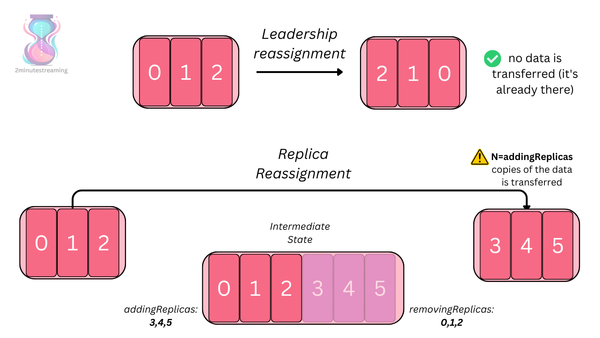
Kafka 101
This is a guest article by Stanislav Kozlovski, an Apache Kafka Committer. If you would like to connect with Stanislav, you can do so on Twitter and LinkedIn. Originally developed in LinkedIn during 2011, Apache Kafka is one of the most popular open-source Apache projects out there. So far it