Strategy: Recompute Instead of Remember Big Data
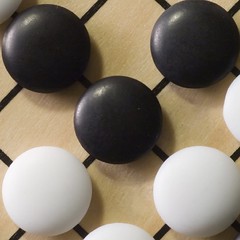
Professor Lance Fortnow, in his blog post Drowning in Data, says complexity has taught him this lesson: When storage is expensive, it is cheaper to recompute what you've already computed. And that's the world we now live in: Storage is pretty cheap but data acquisition and computation are even cheaper.
Jouni, one of the commenters, thinks the opposite is true: storage is cheap, but computation is expensive. When you are dealing with massive data, the size of the data set is very often determined by the amount of computing power available for a certain price. With such data, a linear-time algorithm takes O(1) seconds to finish, while a quadratic-time algorithm requires O(n) seconds. But as computing power increases exponentially over time, the quadratic algorithm gets exponentially slower.
For me it's not a matter of which is true, both positions can be true, but what's interesting is to think that storage and computation are in some cases fungible. Your architecture can decide which tradeoffs to make based on the cost of resources and the nature of your data. I'm not sure, but this seems like a new degree of freedom in the design space.