Google on Latency Tolerant Systems: Making a Predictable Whole Out of Unpredictable Parts
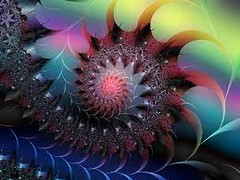
In Taming The Long Latency Tail we covered Luiz Barroso’s exploration of the long tail latency (some operations are really slow) problems generated by large fanout architectures (a request is composed of potentially thousands of other requests). You may have noticed there weren’t a lot of solutions. That’s where a talk I attended, Achieving Rapid Response Times in Large Online Services (slide deck), by Jeff Dean, also of Google, comes in:
In this talk, I’ll describe a collection of techniques and practices lowering response times in large distributed systems whose components run on shared clusters of machines, where pieces of these systems are subject to interference by other tasks, and where unpredictable latency hiccups are the norm, not the exception.
The goal is to use software techniques to reduce variability given the increasing variability in underlying hardware, the need to handle dynamic workloads on a shared infrastructure, and the need to use large fanout architectures to operate at scale.
Two forces motivate Google’s work on latency tolerance:
- Large fanout architectures. Satisfying a search request can involve thousands of machines. The core idea is that small performance hiccups on a few machines causes higher overall latencies and the more machines the worse the tail latency. The statistics of the situation are highly unintuitive. Only 1% of requests will take over a second with a server that has a 1ms average response time and a one second 99th percentile latency. If a request has to access 100 servers, now 63% of all requests will take over a second.
- Resource sharing. One of the surprising aspects of Google’s architecture is they use most of their machines as generalized job execution engines. Not even Google has infinite resources, so they share resources. This also fits where their “the data warehouse is the computer” philosophy. Every node runs linux and participates with a scheduling daemon that schedules work across the cluster. Jobs of various types (CPU intensive, MapReduce, etc) will be running on the same machine that runs a Bigtable tablet server. Jobs are not isolated on servers. Jobs impact each other and those impacts must be managed.
Others try to reduce variability by overprovisioning or running only like workloads on machines. Google makes use of all their resources, but sharing disk, CPU, network, etc, increases variability. Jobs will burst CPU, memory, or network usage, so running a combination of background activities on machines, especially with large fanouts, leads to unpredictable results.
You may think complete and total control would solve the problem, but the more you tighten your grip, the more star systems will slip through your fingers. At a small scale careful control can work, but the disadvantages of large fanout architectures and shared resource execution models must be countered with good design.
Fault Tolerant vs Latency Tolerant Systems
Dean makes a fascinating analogy between creating fault tolerant and latency tolerant systems. Fault tolerant systems make a reliable whole out of unreliable parts by provisioning extra resources. In the same way latency tolerant systems can make a predictable whole out of unpredictable parts. The difference is in the time scales:
- variability: 1000s of disruptions/sec, scale of milliseconds
- faults: 10s of failures per day, scale of tens of seconds
Tolerating variability requires having a fine trigger so the response can be immediate. Your scheduling system must be able to make decisions within these real-time time frames.
Mom and Apple Pie Techniques for Managing Latency
These techniques the “general good engineering practices” for managing latency:
- Prioritize request queues and network traffic. Do the most important work first.
- Reduce head-of-line blocking. Break large requests into a sequence of small requests. This time slices a large request rather than let it block all other requests.
- Rate limit activity. Drop or delay traffic that exceeds a specified rate.
- Defer expensive activity until load is lower.
- Synchronize disruptions. Regular maintenance and monitoring tasks should not be randomized. Randomization means at any given time there will be slow machines in a computation. Instead, run tasks at the same time so the latency hit is only taken during a small window.
Cross Request Adaptation Strategies
The idea behind these strategies is to examine recent behavior and take action to improve latency of future requests within tens of seconds or minutes. The strategies are:
- Fine-grained dynamic partitioning. Partition large datasets and computations. Keep more than 1 partition per machine (often 10-100/machine). Partitions make it easy to assign work to machines and react to changing situations as the partitions can be recovered on failure or replicated or moved as needed.
- Load balancing. Load is shed in few percent increments. Shifting load can be prioritized when the imbalance is severe. Different resource dimensions can be overloaded: memory, disk, or CPU. You can't just look at CPU load because they may all have the same load. Collect distributions of each dimension, make histograms, and try to even out work to machines by looking at std deviations and distributions. Different resource dimensions can be overloaded: memory, disk, or CPU. You can't just look at CPU load because they may all have the same load. Collect distributions of each dimension, make histograms, and try to even out work to machines by looking at std deviations and distributions.
- Selective partitioning. Make more replicas of heavily used items. This works for static or dynamic content. Important documents or Chinese documents, for example, can be replicated to handle greater query loads.
- Latency-induced probation. When a server is slow to respond it could be because of interference caused by jobs running on the machine. So make a copy of the partition and move it to another machine, still sending shadow copies of the requests to the server. Keep measuring latency and when the latency improves return the partition to service.
My notes on this section are really bad, so that's all I have for this part of the talk. Hopefully we can fill it in as more details become available.
Within-request Adaptation Strategies
The idea behind these strategies is to fix a slow request as it is happening. The strategies are:
- Canary requests
- Backup requests with cross-server cancellation
- Tainted results
Backup Requests with Cross-Server Cancellation
Backup requests are the idea of sending requests out to multiple replicas, but in a particular way. Here’s the example for a read operation for a distributed file system client:
- send request to first replica
- wait 2 ms, and send to second replica
- servers cancel request on other replica when starting read
A request could wait in a queue stuck behind an expensive query or a packet could be dropped, so if a reply is not returned quickly other replicas are tried. Responses come back faster if requests sit in multiple queues.
Cancellation reduces the frequency at which redundant work occurs.
You might think this is just a lot of extra traffic, but remember, the goal is to squeeze down the 99th percentile distribution, so the backup requests, even with what seems like a long wait time really bring down the tail latency and standard deviation. Requests in the 99th percentile take so long that the wait time is short in comparison.
Bigtable, for example, used backup requests after two milliseconds, which dramatically dropped the 99th percentile by 43 percent on an idle system. With a loaded system the reduction was 38 percent with only one percent extra disk seeks. Backups requests with cancellations gives the same distribution as an unloaded cluster.
With these latency tolerance techniques you are taking a loaded cluster with high variability and making it perform like an unloaded cluster.
There are many variations of this strategy. A backup request could be marked with a lower priority so it won't block real work. Send to a third cluster after a longer delay.Wait times could be adjusted so requests are sent when the wait time hits the 90th percentile.
Tainted Results - Proactively Abandon Slow Subsystems
- Tradeoff completeness for responsiveness. Under load noncritical subcomponents can be dropped out. It’s better, for example, to search a smaller subset of pages or skip spelling corrections than it is to be slow.
- Do not cache results. You don’t want users to see tainted results.
- Set cutoffs dynamically based on recent measurements.
Related Articles
- Behind The Scenes Of Google Scalability
- The Three Ages Of Google - Batch, Warehouse, Instant
- On HackerNews
- Reliable Digital Communications Systems Using Unreliable Network Repeater Nodes by Paul Baran
- PROBABILISTIC LOGICS AND THE SYNTHESIS OF RELIABLE ORGANISMS FROM UNRELIABLE COMPONENTS by J. von NEUMANN