F1 and Spanner Holistically Compared
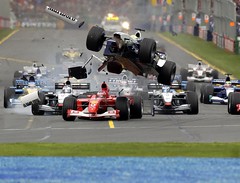
This aricle, F1: A Distributed SQL Database That Scales by Srihari Srinivasan, is republished with permission from a blog you really should follow: Systems We Make - Curating Complex Distributed Systems.
With both the F1 and Spanner papers out its now possible to understand their interplay a bit holistically. So lets start by revisiting the key goals of both systems.
Key Goals of F1′s design
- System must be able to scale up by adding resources
- Ability to re-shard and rebalance data without application changes
- ACID consistency for transactions
- Full SQL support, support for indexes
Spanner’s objectives
- Main focus is on managing cross data center replicated data
- Ability to re-shard and rebalance data
- Automatically migrates data across machinesF1 – An overview
- F1 is built on top of Spanner. Spanner offers support for for features such as – strong consistency through distributed transactions (2PC), global ordering based on timestamps, synchronous replication via Paxos, fault tolerance, automatic rebalancing of data etc.
- To this F1 adds features such as
- Distributed SQL queries with ability to perform joins over external data
- Transactional consistency of indexes
- Asynchronous schema changes
- Uses a new ORM library
F1′s Architecture
- Users interact via a client library
- Any of the servers can receive the SQL query request
- The F1 client’s request goes through a load balancer which is biased towards keeping the latency low. So it tries to forward the request to an F1 server in the same/nearest datacenter unless necessary otherwise.
- The F1 servers are collocated in the same datacenter that house the Spanner’s span-servers.
- The span-servers in turn get their data from the Colossus File System (successor to GFS)
-
- Each span-server works with a storage abstraction called Tablet. Is usually responsible for 100 to 1000 instances of tablets. The tablet’s data is stored on a set of B-Tree like files and a write ahead log. These files reside on CFS.
- Each span-server also implements a single Paxos state machine on top of the tablet.
- F1 servers are mostly stateless. They hold no data and hence and be added or removed easily without requiring any data movement.
- The F1 processes are organized in a master slave fashion. Queries are received by F1 masters and then delegated to the F1 slaves.
- The slave pool membership is maintained by the F1 master.
- Throughput of the system can be increased by increasing the number of F1 masters, F1 slaves and the span-servers.
- Data storage is managed by Spanner.
-
- Spanner partitions data rows into a bucketing abstraction called a directory, (which is a set of contiguous keys that share a common prefix). Ancestry relationships in the schema are implemented using directories.
- Adding a new span-server will cause redistribution of data across Spanner tablets but will not effect any of the F1 servers. Also this process is transparent to the F1 servers.
- Since data is synchronously replicated across multiple datacenters distributed widely geographically the commit latencies are relatively high (50-150 ms).
- The system also has read-only replicas that do not participate in the Paxos algorithm. Read-only replicas are used only for snapshot reads and thus allow segregation of OLTP and OLAP workloads.
Data Model – Hierarchical Schema
- At a logical level F1 has a data model that is similar to an RDBMS. Additionally tables in F1 can be organized into a hierarchy.
- A row corresponding to the root table in the hierarchy is called the root row.
- Rows of child tables related to the root row are stored in one single Spanner directory.
- Client applications declare the hierarchies in database schemas via the INTERLEAVE IN declarations.
- Each row in a directory table with key K, together with all of the rows in descendant tables that start with K in lexicographic order, forms a directory.
- Physically, each child table is clustered with and interleaved within the rows from its parent table.
- The paper goes on to highlight some of the benefits of having a hierarchical schema for both reads and writes. However hierarchical modeling is not mandatory in F1. A flat schema is also possible.
- Indexes in F1 are transactional and fully consistent. Indexes are stored as separate tables in Spanner, keyed by a concatenation of the index key and the indexed table’s primary key.
- It uses two types of physical storage layouts – Local and Global
Query Processing in F1
Its not very surprising to observe that query processing in F1 looks remarkably similar to what we find in the more recent SQL-on-Hadoop solutions such as Cloudera’s Impala, Apache Drill and predecessor shared nothing parallel databases.Lifecycle of a query
- Each query has a query coordinator node. Its the node that receives the SQL query request.
- The coordinator plans the query for execution, receives results from the penultimate execution nodes, performs any final aggregation, sorting, or filtering, and then streams the results back to the client,
- Given that data is arbitrarily partitioned the query planner determines the extent of parallelism needed to minimize the processing time for a query.
- Based on the data required to be processed and scope for parallelism the planner/optimizer may even choose to repartition the qualifying data
Dealing with network latencies
F1′s main data store is Spanner, which is a remote data source. F1 SQL can also access other remote data sources whose accesses involve highly variable network latency.
The issues associated with remote data access (which are issues due to network latency) are mitigated through the use of batching and pipelining across various stages of the query life cycle. Also the query operators are designed to stream as much data as possible to the subsequent stages of the processing pipeline.
And finally….
The F1 system has been managing all AdWords advertising campaign data in production since early 2012. AdWords is a vast and diverse ecosystem including 100s of applications and 1000s of users, all sharing the same database. This database is over 100 TB, serves up to hundreds of thousands of requests per second, and runs SQL queries that scan tens of trillions of data rows per day. Availability reaches five nines, even in the presence of unplanned outages, and observable latency on our web applications has not increased compared to the old MySQL system.