Stuff The Internet Says On Scalability For December 21st, 2018
Wake up! It's HighScalability time:
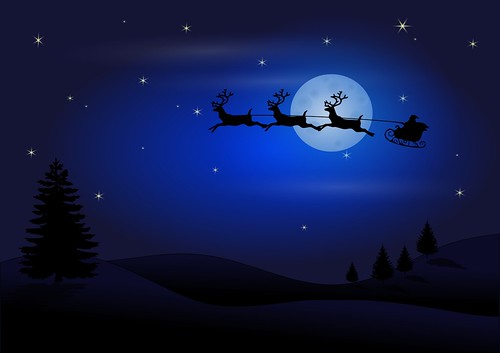
Have a very scalable Xmas everyone! See you in the New Year.
Do you like this sort of Stuff? Please support me on Patreon. I'd really appreciate it. Still looking for that perfect xmas gift? What could be better than a book on the cloud? Explain the Cloud Like I'm 10. And if you know someone with hearing problems they might find Live CC useful.
- 33.5 billion: Pornhub visits; 122 million: miles traveled by Santa; 32,342: government requests to Apple for user data; 10x: faster helicopter design using VR instead of physical models and mockups; 4403: petabytes transferred by Pornhub; 59%: dropped leads on Google AMP; 160: streaming shows now outnumber their traditional-TV counterparts; 80%: machine learning engineers work at Google or Facebook; 25%: adults check phone immediately on waking; 164: iPhone apps made $1 million through in-app subscriptions; 750 petabytes: Backblaze storage;
- Quotable Quotes:
- @ flight radar24: Yesterday was the busiest day of the year in the skies so far and our busiest day ever. 202,157 flights tracked! The first time we've tracked more than 200,000 flights in a single day on
- @swardley: X : What's going to happen in cloud in 2019? Me : Nothing special. 1) Enterprise data centres will continue to close. 2) Cloud will decentralise in terms of provision not power i.e. Amazon will "invade" more of those holdouts with AWS Outpost. 3) Serverless will rocket.
- @odrotbohm: I’ve seen microservice based systems more deranged after 2 years than any 1,5 decades old monolith could ever have been.
- Jason Kehe: Savings of even a few degrees Celsius can significantly extend the lifespan of electronic components; Microsoft reports that, on the ocean floor 117 feet down, its racks stay 10 degrees cooler than their land-based counterparts. Half a year after deployment, “the equipment is happy,” says Ben Cutler, the project’s manager.
- @kellabyte: “Open source” infrastructure companies are a giant shit show right now. Whether it’s database or message queues it’s a really weird combo of licenses and features for hostage. Why? Because nobody knows how to make money. Support contracts aren’t enough.
- Tim Bray: How to talk about [Serverless Latency] · To start with, don’t just say “I need 120ms.” Try something more like “This has to be in Python, the data’s in Cassandra, and I need the P50 down under a fifth of a second, except I can tolerate 5-second latency if it doesn’t happen more than once an hour.” And in most mainstream applications, you should be able to get there with serverless. If you plan for it.
- Jeremy Daly: Compared to building and maintaining your own systems, cloud computing is ridiculously inexpensive, especially when you’re starting out and haven’t achieved significant scale. Don’t waste your developers’ time trying to shave off nickels and dimes from your bill. Focus on creating more value by delivering and iterating on features faster and worry about cost optimizations later.
- Zack Kanter: One day, complexity will grow past a breaking point and development velocity will begin to decline irreversibly, and so the ultimate job of the founder is to push that day off as long as humanly possible. The best way to do that is to keep your ball of mud to the minimum possible size— serverless is the most powerful tool ever developed to do exactly that.
- Dr Ingvars Birznieks: Nerve impulses, it is a binary code - it's just like your mobile phone. It just sends a signal, then it's up to the brain to interpret it. It’s electrical impulses, just like what all our digital devices do, sent in a certain sequence. And that sequence, within one receptor and across different nerve fibres, is a code. And that is what we're trying to break. When we have cracked the full code, we can use it to make prosthetics feel - so that amputees can feel the world again. They will be able to hold the hand of people they love, and feel that touch
- @jamesurquhart: Q: How many "serverless" experts does it take to change a lightbulb? A: Define "lightbulb"…
- Pat Helland: I'm a recovering transactions guy. I love transactions but I think they have their place. Linearizable reads are not always required, it depends on your business needs.
- Jim Handy: The main finding is that the increasing demand for nonvolatile memory will drive total manufacturing equipment revenue used for these tools to rise from an estimated $29M in 2017 to between $517M to $792M by 2028. The details behind this forecast are spelled out in the report.
- @swardley~ No way a 'normal' person would move to AWS .... oh my, Ellison must be desperate to resort to this sort of playground name calling. It's a bit sad.
- Memory Guy: What’s in store for 2019? The industry has already entered the first stages of its collapse, with both NAND flash and DRAM prices falling, and this will continue, thanks to overbuilt capacity, until prices reach cost. This is what always happens when there’s an oversupply. This price collapse will cause DRAM revenues to drop by 35%, and NAND flash revenues to decline by 15%, leading to a decline in overall semiconductor revenues of about 5% year-over-year.
- bunnie: if it looks complicated, that’s because it is. Test jig complexity is correlated with product complexity, which is why I like to say the test jig is the “product behind the product”. In some cases, a product designer may spend even more time designing a test jig than they spend designing the product itself. There’s a very large space of problems to consider when implementing a test jig, ranging from test coverage to operator fatigue, and of course throughput and reliability.
- ksajadi: I've been bitten by service shut downs like this and over the years, here is the "rulebook" I've made for myself to reduce the risk: - Don't use products from startups with unknown, dubious business models that are clearly subsidised by VC money until they "figure out how to monetize"...- Open source doesn't mean it's safe. Very few open source companies have solid business models...- I sometimes even research the VCs backing the founders as well. I feel much safer buying crtical services from a bootstrapped and profitable startup than a well funded one that doesn't have a clear business model.
- Spotify: Without Autoscaler this cluster would probably use 80 nodes all the time, but here we see how the capacity is automatically adjusted to demand (~50 in average).
- Noah Zoschke: The internet-facing segmentapis.com endpoint is an Envoy front proxy that rate-limits and authenticates every request. It then transcodes a #REST / #JSON request to an upstream GRPC request. The upstream GRPC servers are running an Envoy sidecar configured for Datadog stats. The result is API #security , #reliability and consistent #observability through Envoy configuration, not code.
- nodesocket: Developer experience on GCP is vastly superior to AWS. - Pricing on GCP is much easier, no need to purchase reserved instances, figure out all the details and buried AWS billing rules...- GCP projects by default span all regions. It is much easier if you run multiple regions, all services can communicate with all regions...- Custom machine types. With GCE, you simply select the number of cores you need and memory. No trying to decipher the crazy amount of AWS instance types
- Taeer Bar-Yam: Our analysis states that an open system exposed to a structured environment will develop complexity at all scales. By spontaneous variation larger scale complexity will arise, and by the multiscale law of requisite variety the system will adopt that large scale complexity; over enough time all scales will be reached.
- Percona: With HugePages set to 1GB, the higher the number of clients, the higher the comparative performance gain.
- Daniel Abadi: I want to clear up some of the misconceptions and inaccurate assumptions around these latency tradeoffs, and present a deeper (and technical) analysis on how these different approaches to consensus have surprisingly broad consequences on transaction latency. We will analyze the latency tradeoff from three perspectives: (1) Latency for write transactions, (2) Latency for linearizable read-only transactions and (3) Latency for serializable snapshot transactions...The latency debate between unified vs. partitioned consensus is an intricate one. However, it is clear that multi-partition transactions exacerbate the disadvantages of partitioned-consensus transactions in (at least) three dimensions
- rhacker: Personally I think GraphQL's existence, while seemingly blatantly obvious in retrospect, is was what REST should have been. It lets "generic" applications to discover the API and its associated operations. Essentially its also simply a re-do of what Swagger (and a few others) attempted to do, but never really made REST work (imho). Here's a good analogy: Graphql is docker, REST is LXC.
- mismatchpair: I work in the field (DNA nanotechnology) and a question I often get asked is, "When are DNA computers going to replace silicon based computers?" The answer is that that's highly unlikely to happen. They both have their strengths and drawbacks and their own domains. For instance, DNA computing will probably never match the computation speed of silicon based computing since in order for DNA to compute,chemical reactions such as DNA hybridization or dissociation with their complementary counterparts must occur (which is very slow compared to manipulating electron flow). Also, the error rate using DNA is pretty high, e.g., for DNA computing using double-crossover tiles (which is mathematically equivalent to Turing-universal Wang tiles) implementing an XOR logic cellular automata, the best error rate is currently roughly on the order of ~0.1%. Two of the greatest strengths of DNA computation are its energy efficiency and massive parallelism. A microtube containing just 100 ul of DNA solution can have roughly 10^17 or 10^18 strands of DNA working in parallel. Lastly, it may be easier to get computing DNA nanomachines to work /in vivo/ or inside cells as opposed to silicon based nanomachines.
- Pornhub: If you were to start watching 2018’s videos after the Wright brother’s first flight in 1903, you would still be watching them today 115 years later!
- Undrinkable Kool-Aid: That experience at my first job has been consistently repeated at every other job I’ve been at. I’ve started with almost no knowledge about the stack and been up to speed in less than 3 months or so. This is why I always tell people to pick up technology and language agnostic problem solving skills because those are the only skills transferable across stacks. Whatever you can do with one stack you can do with another stack and there is no magic that the stack can provide other than what you personally bring to it.
- Aloha: The article gives the answer early on - the company is still in a phase of change from selling GE Capital - for 20 years they were used to using Capital as a source of excess cash to paper over other temporary market issues elsewhere in the company - they no longer have that ability - so now the cyclical nature of normal business will show directly on their balance sheets and statements.
- pcwalton: I'm not surprised that Microsoft just used "are there any DOM elements over the video?" as a quick heuristic to determine whether scanout compositing can be used. Remember that there is always a tradeoff between heuristics and performance. At the limit you could scan every pixel of each layer to see whether all of them are transparent and cull the layer if so, but that would be very expensive. You need heuristics of some kind to get good performance, and I can't blame Microsoft for using the DOM for that.
- Josh Barratt: I really can’t ‘conclude’ much, this test was tinkering-grade; not science or anything close to it. But I do suspect that right now in AWS, you can generate more brute force load testing requests/second/dollar on Intel than you can ARM. This being a heavily CPU-bound task, that’s in line with what even AWS says about them. It’s still an impressive first outing and I’ll be excited to see what other people do with them.
- InGodsName: I built an Adtech platform on Lambda recently: It's processing 9 billion events per week. 1. We used Firehose which pushed the data to s3 2. Go binary on Lambda transformed the data into Parquet format 3. Used Athena to query this data 4. Using Lambda to adjust the machine learing data based on the ariving data in batches. 5. Using Lambda to query athene/bigquery for data dashboard queries. Again using Go binary for max performance. All this made our platform 10x cheaper
- vhold: A product that actually uses all the features of Oracle would be naturally hilarious. Oracle's documentation is over 500 megabytes compressed. It's an alternative computing reality. Here is a 5559 page book of error codes. Imagine having a physical copy of this on your desk.
- @kellabyte: Most databases have a lot of comparable alternatives that are still painful but doable to change into but here’s the real truth for many of you. If Elastic Search makes a few choice decisions it could fuck a ton of you over with very little options available.
- @jeremy_daly: Unfortunately, not everyone will agree that Fargate, or things like FaaS running on top of your own containers isn’t actually #serverless. There needs to be an agreement from the community as to what meets the criteria.
- Donald Knuth: I am worried that algorithms are getting too prominent in the world. It started out that computer scientists were worried nobody was listening to us. Now I’m worried that too many people are listening.
- Mark Schwartz: Now, a final piece to the puzzle: with the cloud, we can break down even a single digital transaction into its component costs and then work to both optimize those costs and gain insight into our unit economics—not just on a customer-by-customer or transaction-by-transaction basis, but on a digital operation-by-digital operation basis within a transaction. The implications, as I will show, are substantial for finance and IT management.
- Cockroach Labs: AWS outperformed GCP on applied performance (e.g., TPC-C) and a variety of micro-benchmarks (e.g, CPU, network, and I/O) as well as cost.
- Rachel Stephens: We’ve seen a blurring of the definition of ‘serverless’ in the industry as more products are described with the term. As such, we have found “managed services that scale to zero” to be an increasingly useful definition of serverless.
- @rakyll: Scaling to zero is not just a billing concern. Scaling to small and having almost zero entry barrier is the ultimate reasons why managed execution environments win. Having to invest 6 months to a year to learn an execution environment to be confident enough to host your production environment is not a starter.
- @ben11kehoe: Optimizing your Lambda functions requires developer time that can otherwise be spent on creating new features with direct value for customers. Be extra sure the money you'll save by spending that time is worth more than *both* the developer time AND the features. We’re running a fully serverless production system at scale, and even there Lambda is not enough of a cost driver for us to spend much time optimizing them
- Tim Bray: Then consider the fact that you have a finite time budget for software design. If you go serverless, then you you don’t have to design Kubernetes flows or Auto Scaling policies or fleet-health metrics or any of that other stuff. All your design time can be dedicated to, like Werner’s slide says, software that directly addresses business issues. So, given more design time, you’re probably gonna get a better design with serverless. My feeling is, the why of serverless is pretty obvious. It’s the how that’s interesting.
- Robert Kral: Seems like GE was a casualty of the B-school myth that "a good manager can manage anything." Detailed industry knowledge is absolutely essential.
- @ramramanathan1: As an Ex GE employee, it is also a good example when senior leadership comes from primarily Sales or Finance track and get rotatted every 3 yeara.. Minimal domain knowledge. Not sure how many senior leadership knew the energy or healthcare sector in/out
- Duwain Corbell: I worked for GE in Power Services for 37 years...Circa 1990 things changed when six sigma came to GE (I'm not going to knock six sigma the process, it has it's place within organizations). After that time everyone who was on the upward track had to be a six sigma guru more so than being knowledgeable and successful in that business. When I retired in 2009 almost everyone in the Power Services business headquarters was a six sigma black belt. When decisions were made on who took the reins of a department or business the folks making the decisions made management ability, company knowledge and experience subordinate to six sigma (you had to be a black belt!!)...eventually all at the top knew nothing about what went on at the bottom. End of story.....
- Time Bray: I’m pretty convinced, and pretty sure this belief is shared widely inside AWS, that for this sort of control-plane stuff, serverless is the right way to go, and any other way is probably a wrong way. Amazon MQ is a popular service, but how often do you need to wind up a new broker, or reconfigure one? It’d be just nuts to have old-school servers sitting there humming away all the time just waiting for someone to do that. Environmentally nuts and economically nuts. So, don’t do that.
- Conscious Entities: The key point for me is that although the new program is far more general in application, it still only operates in the well-defined and simple worlds provided by rule-governed games. To be anything like human, it needs to display the ability to deal with the heterogenous and undefinable world of real life. That is still far distant (Hassabis himself has displayed an awareness of the scale of the problem, warning against releasing self-driving cars on to real roads prematurely), though I don’t altogether rule out the possibility that we are now moving perceptibly in the right direction.
- Geoff Huston: in times of fundamental change our understanding of the mechanics of the former world just aren't that helpful anymore. A GDP figure that cannot measure the true economic value of freely offered services is not helpful any more. Enterprises now straddle many market sectors and the network effects create fertile incubators that rapidly produce dominant players that assume overarching control within their chosen activity sectors. We navigate our way through this with a public policy framework that attempts to balance the public interest against the self-interest of the private sector. But to have an informed, relevant and effective public policy process we need to understand this changing world. It seems to me that open measurement platforms and open data sets are more important than ever before. We need public measurements that are impartial, accurate, comprehensive and of course unbiased as an essential precondition for the fair and effective operation of markets.
- Robert Graham: If you are building code using gcc on Linux, here are the options/flags you should use: -Wall -Wformat -Wformat-security -Werror=format-security -fstack-protector -pie -fPIE -D_FORTIFY_SOURCE=2 -O2 -Wl,-z,relro -Wl,-z,now -Wl,-z,noexecstack. If you are more paranoid, these options would be: -Wall -Wformat -Wformat-security -Wstack-protector -Werror -pedantic -fstack-protector-all --param ssp-buffer-size=1 -pie -fPIE -D_FORTIFY_SOURCE=2 -O1 -Wl,-z,relro -Wl,-z,now -Wl,-z,noexecstack
- Richard Jones: The consequence seems inescapable – at some point the economic returns of improving the technology will not justify the R&D expenditure needed, and companies will stop making the investments. We seem to be close to that point now, with Intel’s annual R&D spend – $12 billion in 2015 – only a little less than the entire R&D expenditure of the UK government, and the projected cost of doubling processor power from here exceeding $100 billion...The end of this remarkable half-century of exponential growth in computing power has arrived – and it’s important that economists studying economic growth come to terms with this. However, this doesn’t mean innovation comes to an end too. All periods of exponential growth in particular technologies must eventually saturate, whether that’s as a result of physical or economic limits. In order for economic growth to continue, what’s important is that entirely new technologies must appear to replace them. The urgent question we face is what new technology is now on the horizon, to drive economic growth from here.
- Russ Olsen: I spent the next weekend hacking together a version of the system that packaged everything in a single process. The difference was dramatic. Simple pictures now drew more or less instantaneously while more complex ones would only take one sip of coffee to finish. Monday morning I demo'ed my hacked up version over and over: First to my boss and then to my boss's boss and then to his boss and then to a whole assortment of higher ups. And then all Hell broke lose. Many of those boss's boss's bosses were seriously pissed at me, though no one could or would articulate exactly why. Some of my co-workers started treating me like I had contracted an infectious disease. Slowly, I figured out that I had jumped into the middle of some complex interdepartmental power struggle. In my own clueless way I hadn't sped up the graphics so much as I had supplied a war winning weapon to one organizational faction and the other factions were not happy.
- Serverless Computing: One Step Forward, Two Steps Back. Let's assume serverless wonderfulness has already been excessively covered. What are the two steps back?
- Joseph M. Hellerstein: After 10 years of people writing cloud programs in legacy sequential languages like Java, the public cloud providers are finally proposing a programming model for the cloud. They are calling it Serverless Computing, or more descriptively “Functions as a Service” (FaaS). As an interface to the unprecedented potential of the cloud, FaaS today is a disappointment. Current FaaS offerings do provide a taste of the power of autoscaling, but they have fatal flaws when it comes to the basic physics of the cloud: they make it impossible to do serious distributed computing, and crazy expensive/slow to work with data at scale. This is not a roadmap for harnessing the creativity of the developer community.
- spullara: Going through the article I think they made some mistakes in analysis and also miscategorized some features. 1) Limited Lifetimes, Stickiness: These is good and leads to good design when you scale beyond a single server. It prepares you for dealing with the reality that if you want to run a service 24/7 with no downtime you need to prepare for things like different versions being in production, failures, and stale caches. 2) I/O Bottlenecks: If you are parallelizing your workloads you will actually see more aggregate bandwidth than you could scale up quickly with normal server hardware. Sure a single Lambda might not have the full amount but you can run 1000 of them at once. 3) Communication through slow storage: This is not entirely true, you can call another Lambda directly but yes you can't access a particular instance. That is a good thing. Designing systems where you need to return to the same instance is an anti-pattern. 4) No Specialized Hardware: I don't expect this to be a limitation for long. There is no reason why you couldn't ask for specialized hardware in the definition of a Lambda and the scheduler take care of it. 5) Faas is a datashipping arch: Not even true today. Lambda lets you move the computation to the data like they are with CloudFront Lambda, S3 Batch and Snowball Edge Compute. It is in fact easier to execute the code near the data when encapsulated in this way. 6) FaaS Stymies Dist Computing: Maybe they have applications that can afford to fail at any point and not recover but keeping your global state in a distributed data storage system is the right thing to do generally, not just with Lambda. Might not work for HPC but it generally doesn't need to be reliable in the same way applications do.
- ciconia: for me the biggest shortcoming in serverless is that it is actually a poor fit for modern interactive web apps (and apps in general) with a constantly changing state. Technologies such as HTTP/2, websocket and SSE are clearly pointing in the direction of long-running client-server interaction, yet current serverless solutions have (to the best of my knowledge) no answer for that. I think the big challenge is in how to do serverless computing with long-running processes, in a way that solves isolation and scalability at the same time.
- bartread: This absolutely kills serverless for me: we are moving into a world where real-time interaction and updates are critical for serving our customers. HTTP/2, websockets, and SSE bring various benefits.
- scarface74: That’s kind of the point. People act like lambda is some weird thing that completely changes your architecture. Even when I’m hosting things on VMs, I still consider them as disposable. I don’t store state on them, logs are all sent to a central store like Cloudwatch or ElasticSearch, if a health check fails, autoscaling just kills the instance and initiates another one based on a custom prebaked image and a startup script, etc. Long lived data is stored in a database and cached with either Memcache or Redis
- Videos from Seattle '18 KubeCon are now available.
- In the distant past we've talked about Cloud Programming Directly Feeds Cost Allocation Back Into Software Design. Now that's become reality. Micro-Optimization: Activity-Based Costing for Digital Services?: consider that each customer transaction is typically handled by multiple pieces of code, called microservices. For example, when you buy something online, your transaction is probably delegated to a microservice that looks up your customer information, another that checks inventory, another that processes your credit card payment, and another that tells the warehouse to pick and package the item. Some of these microservices might have been created by your technologists in-house; some might be services provided by third parties. Each of these microservices uses serverless compute—and you can find out the cost of executing that microservice. Putting all these pieces together you can say that a digital transaction requires a number of activities, and you can break the cost of a transaction into the cost of its component activities. You can do activity-based costing, that is, even for things that are internal to a computing infrastructure!
- It's true, there's no individual competitive advantage for Microsoft to continue developing their own Edge browser. Moving to chrome is just good business, as they say. But as we've seen with SQLite, ubitquity is it's own risk. SQLite is everywhere because it's easy to use and does the job. Thank network effects for the rest. The problem is when SQLite has serious vulnerabilities—remote code execution—then the entire ecosystem is immediately at risk. Monocultures aren't robust, yet the winner take all nature of tech over time tends to produce monocultures. While Microsoft benefits from their decision, herd immunity would benefit from more diversity. Innoculate now. There are no bulkheads for ubiquity.
- A good DockerCon EU 2018 Summary.
- How we built Globoplay’s API Gateway using GraphQL. Globoplay is a video streaming platform. In mid 2018, we had two backends for frontends (BFF) doing very similar tasks: One for web, and another for iOS, android and TV. As much as I love the “backend for frontend” idea (and how cool it sounds), we could not keep the current architecture. Not only because of the reasons I just said, but because each BFF was serving slightly different content to its clients while the business team started to ask for something new: Ubiquity among all clients. the more I reviewed everything we needed to support, the more GraphQL started to make sense. While TVs need a big program poster, mobiles need a small one. We need to show exactly the same video duration among all clients. TVs should provide detailed information about each program, but iOS and android could show only a poster + program title.
They run a nodejs apollo server that is hosted in their own infrastructure. Result: Now the teams are enjoying the fact that they work with this API Gateway that allow them to ask for whatever they need to do their job. Business team is excited because we provided support for the ubiquity among all clients that they were asking for. - A fun and informative talk on Stripe's move to k8s (cron jobs, ML, some http services) and Envoy (service-to-service networking). Keynote: High Reliability Infrastructure Migrations - Julia Evans, Software Engineer, Stripe. Stripe's infrastructure doesn't just optimize for performance, it optimizes for reliability and security, which is what you want from a payments company. Focus on knowing the design of the components you use. Use gamedays to cause problems on purpose using known failure conditions. Uncovered a lot of problems. Goal is to have every incident only one time. Envoy problems (request timeouts, connection timeouts, slow requests, thundering herd) were related to connection pooling issues from using HTTP/1 instead of HTTP/2. Tell your coworkers what you learned through incident reports. Make incremental changes. Don't expose k8s to developers, this reduces their cognitive load. Develop clear interface boundaries. Yaml sucks, let developers call functions to define k8s services in a language called Skylark that generates k8s configuration. Always have a rollback plan. Reliability is a team sport that you all do together. It's OK not to start out as an expert, but the people on the ream need to become experts. Understand the system and the failure modes. Become an engine of learning. Explain to others what went wrong. Adopting software like this is a long term investment. Managers need to create a space for the team to fix issues.
- Do you always need a cloud? Nope, a lot can be done on hardware these days. Google's Top Shot runs on the Pixel 3 as a background process using a hardware-accelerated MobileNet-based single shot detector (SSD). It looks at : 1) functional qualities like lighting, 2) objective attributes (are the subject's eyes open? Are they smiling?), and 3) subjective qualities like emotional expressions. A layered Generalized Additive Model (GAM) provides quality scores for faces and combine them into a weighted-average “frame faces” score.
- Envoy Proxy at Reddit: Recently, we rolled out Envoy as our service-to-service L4/L7 proxy as part of our efforts to address these new and ever-growing needs for developing and maintaining stable production services...Today, the majority of our services run on AWS EC2 instances in AutoScaling Groups...A great deal of Envoy’s advanced feature set that differentiated it for us was its L7 control...Performance-wise, Envoy has had no measurable impact on our service latencies compared to HAProxy. HAProxy is still running as a sidecar to facilitate quick emergency rollbacks during the holiday season while engineering resources are a bit thin, so we haven’t yet been able to measure resource utilization impact on our hosts. Envoy has also provided more observability at the network layer for us, especially after we enabled the Thrift filter on a few internal services. Thanks to the filter instrumentation, Envoy has started to provide request and response metrics that we didn’t have access to before without any application code changes. These small but impactful improvements and the overall operational stability have given us the confidence to continue pursuing our larger service-mesh roadmap for 2019, with Envoy as the engine to power it.
- Scaling Cash Payments in Uber Eats: The product proposal was novel: leverage our restaurant-partners as a distributed network of cash drop-off locations. Delivery-partners with an outstanding cash balance from previous trips would be dispatched to participating restaurants and pay for the order in cash, agnostic to the eater’s payment type. To avoid unnecessary friction during pick-up, the payment amount would equal the order amount, as receiving an amount that differed could create confusion for a restaurant cashier. Restaurants would keep all cash earnings and benefit from the immediate payment. Uber would cover its delivery booking fees by deducting them from subsequent digital orders. This mechanism works well because it scales in present and future Uber Eats markets and makes cash payments a regular part of the delivery experience by integrating them with each trip. Our next challenge was to ensure that restaurant arrears collection could function offline. Fortunately, we built offline support into our new driver app to cover a number of use cases, such as when a driver ends a trip in an area with limited network coverage. This feature dovetailed nicely with our requirements for cash payments.
- What could up to 3x better compression do for you? Facebook detailed 5 use cases from their experience with Zstandard. Use case 1: Very large payloads. Example: write-once and read-never payloads as backing up development servers and as in large payloads for distributing packages to the fleet. Changes: multi-threaded compression, large window sizes, and automatic level determination. Result: an improvement of approximately 10 percent in compression ratio for equivalent or better transmission time. Use case 2: Warehouse. Large data stored in Hadoop and accessed in batches. Changes: effectively make use of megabytes of history. Result: mproved compression ratio and speed by double-digit percentages and reduced data transformations. Use case 3: Compressed filesystems. Resule: For SquashFS, a popular read-only compressed filesystem, 2x faster reads, and the packages were 15 percent smaller. For Btrfs, a modern copy-on-write filesystem, developers perceived 3x more storage, on average, without a noticeable drop in performance. Use case 4: Databases. Hybrid compression: In MyRocks deployments (UDB — the biggest database tier at Facebook, and Facebook Messenger), we decided to use zstd in the bottommost level (where most data files are placed) and to use LZ4 for other levels. LZ4 is faster for compression, which helps to keep up with write ingestion. Zstd in the bottommost level is able to save even more space than zlib, while preserving excellent read speeds. Dictionary Compression: A dictionary makes it possible for the compressor to start from a useful state instead of from an empty one, making compression immediately effective, even when presented with just a few hundred bytes. The dictionary is generated by analyzing sample data, presuming that the rest of the data to compress will be similar. The zstd library offers dictionary generation capabilities that can be integrated directly into the database engine. Result: database can now serve more queries and compact data even further. Use case 5: Managed Compression. There are many other contexts and applications in which dictionary-based compression would provide large wins. Dictionary compression has a successful implementation story within Facebook’s massive messaging infrastructure. Result: cut the service’s storage appetite. managed compression, is split into halves: an online half and an offline half. It has been deployed at Facebook and relies on several core services. One important use case for managed compression is compressing values in caches. Traditional compression is not very effective in caches, since each value must be compressed individually. Managed compression exploits knowledge of the structure of the key (when available) to intelligently group values into self-similar categories. It then trains and ships dictionaries for the highest-volume categories. The gains vary by use case but can be significant. Our caches can store up to 40 percent more data using the same hardware. Managed compression is now used by hundreds of use cases across Facebook’s infrastructure. Over and over, we’ve seen it deliver consistent, significant compression ratio improvements (on average, 50 percent better than the regular compression methods it replaced).
-
Data Universe videos are now available.
- Going Head-to-Head: Scylla vs Amazon DynamoDB: DynamoDB failed to achieve the required SLA multiple times, especially during the population phase. DynamoDB has 3x-4x the latency of Scylla, even under ideal conditions. DynamoDB is 7x more expensive than Scylla. Dynamo was extremely inefficient in a real-life Zipfian distribution. You’d have to buy 3x your capacity, making it 20x more expensive than Scylla. Scylla demonstrated up to 20x better throughput in the hot-partition test with better latency numbers
- Scale By The Bay 2018: Pat Helland, Keynote III: Mind Your State for Your State of Mind. State means different things. Session state: is across running things. Stateful sessions remembers stuff; Stateless doesn't remember on the session. Durable state: is across failures. Stuff is remembered when you come back later. Most stateful computing comprises microservices with stateless interfaces. Mircroservices need partitioning, failures, and rolling update which means stateful sessions are problematic. Microservices may call other microservices to read data or get stuff done. Transactions across stateless calls usually aren't supported in microservice solutions. Microservice -> no server-side session state -> no transactions across calls -> no transactions across objects. Coordinated changes use the careful replacement technique. Each update provides a new version of the stuff with a single identity. Complex content within the new version may include many things including outgoing/incoming messages. Different applications demand different behaviours from durable state. Do you want it right ("read you writes") or do you want it right now (bounded by fast SLA)? Humans usually prefer right now. Many app solution based on object identity may be tolerant of stale versions. Immutable objects can provide the best of both by being right and right now.
- Datadog's 8 emerging trends in container orchestration: Of the organizations running containers in GCP, more than 85 percent orchestrate workloads with Kubernetes; In the Azure cloud, which has offered a managed Kubernetes service for about two years, roughly 65 percent of organizations using containers also run Kubernetes; One third of our customers using containers now use Kubernetes; in the months after EKS launched, we saw a significant uptick in the number of AWS container organizations running Kubernetes; Fargate has already been adopted by 6 percent of AWS organizations using containers; more than 40 percent of organizations run Kubernetes or ECS when they first start using containers, with smaller numbers of organizations deploying containers with Fargate, Nomad, or Mesos from the start; we see widespread deployment of container images for common infrastructure technologies like NGINX, Postgres, and Elasticsearch, with NGINX appearing in two thirds of Kubernetes environments; our data shows that Kubernetes pods tend to run slightly fewer containers than ECS or Fargate tasks; Kubernetes nodes tend to run large numbers of single-process containers, whereas ECS nodes run fewer containers, some of which run multiple processes; At the median Kubernetes organization, each host runs about 14 containers over a one-hour sampling window, versus just seven containers in the median ECS organization.
- Facebook's HyperLogLog Functions: Today, we are sharing the data structure used to achieve these improvements in speed. We will walk through newly open-sourced functions with which we can further save on computations. Depending upon the problem at hand, we can achieve speed improvements of anywhere from 7x to 1,000x.
- Trill: a high-performance one-pass in-memory streaming analytics engine from Microsoft Research. It can handle both real-time and offline data, and is based on a temporal data and query model. Trill can be used as a streaming engine, a lightweight in-memory relational engine, and as a progressive query processor (for early query results on partial data). Trill can handle a trillion events per day. Trill’s high performance across its intended usage scenarios means users get results with incredible speed and low latency. For example, filters operate at memory bandwidth speeds up to several billions of events per second, while grouped aggregates operate at 10 to 100 million events per second.
- AMBROSIA (Actor-Model-Based Reliable Object System for Internet Applications): a new open source project from Microsoft Research. Rather than placing the burden on application developers to build fault-tolerance into their systems from scratch, AMBROSIA provides a general-purpose distributed programming platform that automatically handles failure and lets the developer focus on the core logic of their application. We call this property of AMBROSIA “virtual resiliency”. Virtual resiliency is achieved by running your application code in an AMBROSIA immortal (see diagram below), which handles checkpointing, logs all communications going into and out of your application, and writes them to storage.
- umpywren: serverless linear algebra: We present numpywren, a system for linear algebra built on a serverless architecture. We also introduce LAmbdaPACK, a domain-specific language designed to implement highly parallel linear algebra algorithms in a serverless setting. We show that, for certain linear algebra algorithms such as matrix multiply, singular value decomposition, and Cholesky decomposition, numpywren's performance (completion time) is within 33% of ScaLAPACK, and its compute efficiency (total CPU-hours) is up to 240% better due to elasticity, while providing an easier to use interface and better fault tolerance
- Scaling Multi-Agent Reinforcement Learning: This blog post introduces a fast and general framework for multi-agent reinforcement learning. We’re currently working with early users of this framework in BAIR, the Berkeley Flow team, and industry to further improve RLlib. To show the importance of these optimizations, in the below graph we plot single-core policy evaluation throughout vs the number of agents in the environment. For this benchmark the observations are small float vectors, and the policies are small 16x16 fully connected networks. We assign each agent to a random policy from a pool of 10 such policy networks. RLlib manages over 70k actions/s/core at 10000 agents per environment (the bottleneck becomes Python overhead at this point). When vectorization is turned off, experience collection slows down by 40x: