Stuff The Internet Says On Scalability For October 4th, 2019
Wake up! It's HighScalability time:
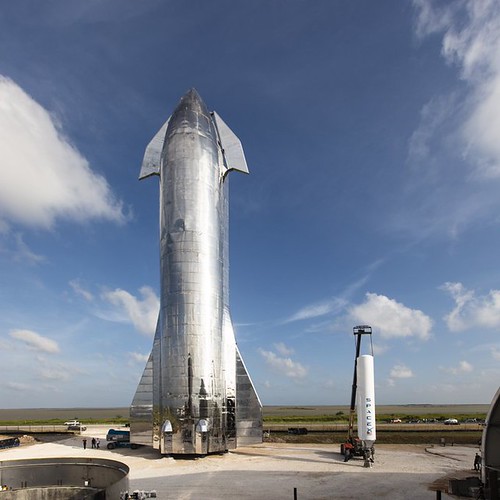
SpaceX ready to penetrate space with their super heavy rocket. (announcement)
Do you like this sort of Stuff? I'd greatly appreciate your support on Patreon. And I wrote Explain the Cloud Like I'm 10 for all who want to understand the cloud. On Amazon it has 57 mostly 5 star reviews (135 on Goodreads). Please recommendint it. They'll love you even more.
Number Stuff:
- 94%: lost value in Algorand cryptocurrency in first three months.
- 38%: increase in machine learning and analytics driven predictive maintenance in manufacturing in the next 5 years.
- 97.5%: Roku channels tracked using Doubleclick. Nearly all TVs tested contact Netflix, even without a configured Netflix account. Half of TVs talk to tracking services.
- 78: SpaceX launches completed in 11 years.
- 70: countries that have had disinformation campaigns.
- 99%: of misconfigurations go unreported in the public cloud.
- 12 million: reports of illegal images of child sex abuse on Facebook Messenger in 2018.
- 40%: decrease in trading volume on some major crypto exchanges last month.
- 2016: year of peak global smartphone shipments.
- 400,000: trees a drone can plant per day. It fires seed missiles into the ground. In less than a year the trees are 20 inches tall.
- 14 million: Uber rides per day.
- 90%: large, public game companies (Epic, Ubisoft, Nintendo) run on the AWS cloud.
- $370B: public cloud spending by 2022.
- C: language with the most curse words in the comments.
- 45 million: DynamoDB TPS. Bitcoin? 14 transactions per second.
- 50,000: Zoho requests per second.
- 700 nodes: all it takes for Amazon to cover Los Angeles with a 900 MHz network.
- One Quadrillion: per day real-time metrics at Datadog.
Quotable Stuff:
- @Hippotas: The LSM tree is the workhorse for many modern data management systems. Last week we lost Pat O’Neil, one of the inventors of the LSM tree. Pat had great impact in the field of databases (LSM, Escrow xcts, LRU-K, Bitmap Indices, Isolation levels, to name few). He will be missed.
- @ofnumbers: pro-tip: there is no real point in using a blockchain - much less a licensed "distributed ledger" - for internal use within a silo'ed organization. marketing that as a big innovative deal is disingenuous.
- @amcafee: More on this: US digital industries have exploded over the past decade (and GDP has grown by ~25%), yet total electricity use has been ~flat. This is not a coincidence; it's cause and effect. Digitization makes the entire economy more energy efficient.
- ICO: GDPR and DPA 2018 strengthened the requirement for organisations to report PDBs. As a result, we received 13,840 PDB reports during 2018-19,an increase from 3,311 in 2017-18.
- Jeanne Whalen: Demand for labeling is exploding in China as large tech companies, banks and others attempt to use AI to improve their products and services. Many of these companies are clustered in big cities like Beijing and Shanghai, but the lower-tech labeling business is spreading some of the new-tech money out to smaller towns, providing jobs beyond agriculture and manufacturing.
- IDC: Overall, the IT infrastructure industry is at crossing point in terms of product sales to cloud vs. traditional IT environments. In 3Q18, vendor revenues from cloud IT environments climbed over the 50% mark for the first time but fell below this important tipping point since then. In 2Q19, cloud IT environments accounted for 48.4% of vendor revenues. For the full year 2019, spending on cloud IT infrastructure will remain just below the 50% mark at 49.0%. Longer-term, however, IDC expects that spending on cloud IT infrastructure will grow steadily and will sustainably exceed the level of spending on traditional IT infrastructure in 2020 and beyond.
- Brian Roemmele: I can not overstate enough how important this Echo Network will become. This is Amazon owning the entire stack. Bypassing the ancient cellular network concepts and even the much heralded 5G networks.
- Ebru Cucen~ Why serverless? Everyone from management to engineering wanted serverless. everyone wanted serverless. It was the first in a project everyone was on-board.
- Jessica Kerr: Every piece of software and infrastructure that the big company called a capital investment, that they value because they put money into it, that they keep using because it still technically works -- all of this weight slows them down.
- @Obdurodon: Saw someone say recently that bad code crowds out good code because good code is easy to change and bad code isn't. It's not just code. (1/2)
- Paul Nordstrom: spend more time talking to your users about how they would use your system, show your design to more people, you know, just shed the ego and shed this need for secrecy if you can, so that you get a wider spectrum of people who can tell you, I’m gonna use it like this. And then, when you run into the inevitable problem, you know, then you just have to, that having done the work that did before, your system will be cleaner design, you’ll have this mathematical model.
- @shorgio: Hotels are worse for long term rent prices. Airbnb keeps hotel profits in check. Without Airbnb, hotel margins grow so there is an incentive to rezone to build more hotels, which can’t be converted back into actual homes
- @techreview: In January, WhatsApp limited how often messages can be forwarded—to only five groups instead of 256—in an attempt to slow the spread of disinformation. New research suggests that the change is working.
- @dhh: The quickest way to ruin the productivity of a small company is to have it adopt the practices of a large company. Small companies don’t just need the mini version of whatever maxi protocol or approach that large companies use. They more often than not need to do the complete opposite.
- @random_walker: When we watch TV, our TVs watch us back and track our habits. This practice has exploded recently since it hasn’t faced much public scrutiny. But in the last few days, not one but *three* papers have dropped that uncover the extent of tracking on TVs. Let me tell you about them.
- @lrvick: The WhatsApp backdoor is now public and official. I have said this many times: there is no future for privacy or security tools that are centralized or proprietary. If you can't decentralize it some government will strongarm you for access.
- Rahbek: The global pattern of biodiversity shows that mountain biodiversity exhibits a visible signature of past evolutionary processes. Mountains, with their uniquely complex environments and geology, have allowed the continued persistence of ancient species deeply rooted in the tree of life, as well as being cradles where new species have arisen at a much higher rate than in lowland areas, even in areas as amazingly biodiverse as the Amazonian rainforest
- @mipsytipsy: key honeycomb use cases. another: "You *could* upgrade your db hardware to a m2.4xl. Or you could sum up the db write lock time held, break down by app, find the user consuming 92% of all lock time, realize they are on your free tier...and throttle that dude."
- Dale Rowe: The internet is designed as a massive distributed network with no single party having total control. Fragmenting the internet (breaking it down into detached networks) would be the more likely result of an attempt. To our knowledge this hasn’t been attempted but one would imagine that some state actors have committed significant research to develop internet kill switches.
- @cloud_opinion: Although we typically highlight issues with GCP, there are indeed some solid products there - have been super impressed with GKE - its solid, priced right and works great. Give this an A+.
- David Wootton: This statement seems obvious to us, so we are surprised to discover that the word competition was a new one in Hobbes’ time, as was the idea of a society in which competition is pervasive. In the pre-Hobbesian world, ambition, the desire to get ahead and do better than others, was universally condemned as a vice; in the post-Hobbesian world, it became admirable, a spur to improvement and progress.
- John Currey: What's really nice with the randomization is that every node is periodically checking every other node. They're not checking that particular node so often, but collectively, all the nodes are still checking all of the other nodes. This greatly reduces the chance of a particular node failure not being discovered
- E-Retail Expansion Report: With over $140 billion in ecommerce sales to consumers in other countries, some U.S. retailers are thinking globally. But only half of U.S. retailers in the Internet Retailer Top 1000 accept online orders from consumers in other countries. The most common way Top 1000 e-retailers sell to shoppers in foreign nations is by accepting orders on their primary websites and then shipping parcels abroad. However, only 46.4% of these retailers ship to the United Kingdom, and 43.4% ship to Japan, two of the largest ecommerce markets. Larger retailers are more likely than smaller ones to ship to foreign addresses, with 70.1% of Top 1000 retailers ranked Nos. 1-100 shipping outside of North America, compared to only 48.4% of those ranked 901-1000
- Ruth Williams: The finding that a bacterium within a bacterium within an animal cell cooperates with the host on a biosynthetic pathway suggests the endosymbiont is, practically speaking, an organelle.
Useful Stuff:
- WhatsApp experiences a connection churn of 600k to 1.5 million connections per second. WhatsApp is famous for using very few servers running Erlang in their core infrastructure. With the 2014 Facebook acquisition a lot has changed, but a lot hasn't changed too. Seems like they've kept that same Erlang spirit. Here's a WhatsApp update on Scaling Erlang Cluster to 10,000 Nodes.
- Grew from 200m users in 2013 to 1.5 billion in 2018 so they needed more processing power as they add more features and users. In the process they were moving from SoftLayer (IBM, FreeBSD, Erlang R16) to Facebook's infrastructure (Open Compute, Linux, Erlang R21) after the 2014 acquisition. This required moving from large powerful dual socketed servers to tiny blades with a max of 32 gig of RAM. Facebook's approach is to pack a lot of servers into a tiny space. Had to move to Erlang R21 to get the networking performance and connection density on Linux that they had on FreeBSD. Now they have a combination of old and new machines in a single cluster and they went from just a few servers to 10,000 smaller Facebook servers.
- An Erlang cluster is a mesh. Every node connects to every other node in the cluster. That's a lot of connections. Not a problem because a million users are assigned to a single server so adding 10,000 connections to a server is not a big deal. They put 1500 nodes in a single cluster with no connection problems. The problem is discovery, when a user on one server talks to another user on a different server. They use two process registries. One is centralized for high rate registrations that acts as a session manager for phones connecting to servers. Every time a phone connects it registers itself in a session manager. A second process registry uses pg2 and globally replicated state for rare changes. A phone connects to an erlang server called a chat node. When a phone wants to connect to another phone it asks a session manager a server the phone is connected to. They have a connection churn of 600k to 1.5 million connections per second. pg2 is used for service discovery mapping which servers to services. Phone numbers are hashed to servers. Meta-cluster are clusters of services: chat, offline, session, contacts, notifications, groups—that are mesh connected as needed. Even with all their patches they can't scale pg2 to 1500 nodes. Clusters are connected with wandist, a custom service.
- It wasn't easy to move from FreeBSD to Linux, kqueue is awesome and epoll is not as awesome. Erlang R21 supports multiple poll sets so it leverages existing Linux network capabilities. With kqeueu you can update a million file descriptors with a single call. With epoll you would need a million individual kernel calls. Given recent security concerns system calls are not as cheap as you would like them to be.
- As in 2014 most scalability problems are caused by a lack of concurrency, which means locking bottlenecks. Bottlenecks must be identified and fixed. Routing performance was a problem. Moving to multiple datacenters meant they had to deal with long range communications which added more latency. Some bottlenecks were found and overcome by adding my concurrency and more workers. Another problem is SSL is really slow on erlang.
- There are also lots of Erlang bugs they had to fix. The built-in tools are great for fixing problems. First line is using the built-in inspection facilities. For distributed problems they use MSACC - microstate accounting with extra accounting turned on. Lock Counting is a tool to find locks. Since Erlang is open source you can change code to help debugging.
- Erlang is getting better so many of the patches they made originally are no longer needed. For example, Erlang introduced off heap messages to reduce garbage collection pressure. But as WhatsApp grows they run into new bottlenecks, like the need for SSL/TLS handshake acceleration. WhatsApp adds more monitoring, statistics, wider lock tables, more concurrency. Some of these patches will go upstream, but many never will. The idea is because Erlang is open source you can make your own version. They are now trying to be more open and push more of their changes upstream.
- eBay created a 5000 node k8s cluster for their cloud platform. Here's how they made it workish. Scalability Tuning on a Tess.IO Cluster.
- To achieve the reliability goal of 99.99%, we deploy five master nodes in a Tess.IO cluster to run Kubernetes core services (apiserver, controller manager, scheduler, etcd, and etcd sidecar, etc). Besides core services, there are also Tess add-ons in each node that expose metrics, set up networks, or collect logs. All of them are watching resources they care about from the cluster control plane, which brings additional loads against the Kubernetes control plane. All the IPs used by the pod network are global routable in the eBay data center. The network agent on each node is in charge of configuring the network on host.
- There were problems: Failed to recover from failures on cluster with: 5k nodes, 150k pods; Pod scheduling is slow in a large cluster; Large list requests will destroy the cluster; Etcd keeps changing leaders.
- There were solutions, but it took a lot of work. If you aren't eBay it might be difficult to pull off.
- The Evolution of Spotify Home Architecture. This is a common story these days. The move from batch to streaming; the move from running your own infrastructure to moving to the cloud; the move from batch recommendations to real-time recommendations; the move from relative simplicity to greater system complexity; the move from more effort put into infrastructure to more effort being put into product.
- At Spotify, we have 96 million subscribers, 207 million monthly active users, we've paid out over €10 billion to rights holders. There are over 40 million songs on our platform, over 3 billion playlists on our service, and we're available in 79 markets.
- We were running a lot of Hadoop jobs back in 2016. We had a big Hadoop cluster, one of the largest in Europe at the time, and we were managing our services and our databases in-house, so we were running a lot of things on-premise. Experimentation in the system can be difficult. Let's say you have a new idea for a shelf, a new way you want to make a recommendation to a user, there's a lot in the system that you need to know about to be able to get to an A/B test. There’s also a lot of operational overhead needed to maintain Cassandra and Hadoop. At that time we were running our own Hadoop cluster, we had a team whose job it was just to make sure that that thing was running.
- We started to adopt services in 2017, this is at the time where Spotify was investing and moving to GCP. What are some of the cons of this? You saw that as we added more and more content, as we added more and more recommendations for the users, it would take longer to load home because we are computing these recommendations at the request spot. We also saw that since we don't store these recommendations anywhere, if for some reason the request failed, the user would just see nothing on the homepage, that's a very bad experience.
- In 2018, Spotify is investing heavily in moving the data stack also to Google Cloud. Today, we're using a combination of streaming pipelines and services to compute recommendations on home that you see today. What's the streaming pipeline? We are now updating recommendations based on user events. We are listening to the songs you have listened to, the artists you have followed, and the tracks you have hearted, and we make decisions based on that. We've separated out computation of recommendations and serving those recommendations in the system. What are some of the cons? Since we added the streaming pipelines into this ecosystem, the stack has just become a little bit more complex. Debugging is more complicated, if there is an incident on your side, you have to know whether it's the streaming pipeline, or your service, it's the logic, or it is because Bigtable is having an issue.
- Datadog: a Real-Time Metrics Database for One Quadrillion Points/Day:
- The key challenge I think for any of us who have worked with S3 is it's great at this like bulk gerbil storage where you just want the blob back. But for any type of high throughput, definitely with our real-time requirements, S3 in and of itself is not going to ever perform well enough. What this tells us is S3's going to be great for a long-term gerbil store, but to really scale this, we need to do something faster. That turns into the question of what.
- Just starting with everyone, let's just say, everyone who wants to do things fast and is just going in-memory databases today, what does the math of that look like? 300 terabytes, that's 80 x 1e.32xlarge for a month. That takes it to $300,000 for a month. Now you're getting into a really expensive system for one customer. This is with no indexes or overhead.
- we use LevelDB for SSD storage where it's very high-performing. DRAM is for in-memory. We like Cassandra, where we can trust it to horizontal scaling, more mid-performance. We use Rocks DB and SQLite for when we want a lot of flexibility in types of queries that we want to run.
- The other thing is, particularly when we're storing stuff in memory, what we found is that there is no real substitute for just picking the right data structures and just storing them in memory. We use a lot of Go code just using the right indexes and the right clever patterns to store anything else. The key takeaway, this is a lot of data, but what we've found is to do this well and at scale, it's a very hybrid approach to traditional systems.
- Attention. HTTP/3 is a thing. HTTP/3: the past, the present, and the future:
- Chrome, curl, and Cloudflare, and soon, Mozilla, rolling out experimental but functional, support for HTTP/3
- instead of using TCP as the transport layer for the session, it uses QUIC, a new Internet transport protocol, which, among other things, introduces streams as first-class citizens at the transport layer. QUIC streams share the same QUIC connection, so no additional handshakes and slow starts are required to create new ones, but QUIC streams are delivered independently such that in most cases packet loss affecting one stream doesn't affect others. This is possible because QUIC packets are encapsulated on top of UDP datagrams.
- QUIC also combines the typical 3-way TCP handshake with TLS 1.3's handshake. Combining these steps means that encryption and authentication are provided by default, and also enables faster connection establishment.
- Processing 40 TB of code from ~10 million projects with a dedicated server and Go for $100:
- I have 12 million or so git repositories which I need to download and process.
- This worked brilliantly. However the problem with the above was firstly the cost, and secondly lambda behind API-Gateway/ALB has a 30 second timeout, so it couldn’t process large repositories fast enough. I knew going in that this was not going to be the most cost effective solution but assuming it came close to $100 I would have been willing to live with it. After processing 1 million repositories I checked and the cost was about $60 and since I didn’t want a $700 AWS bill I decided to rethink my solution.
- How does one process 10 million JSON files taking up just over 1 TB of disk space in an S3 bucket?
- The first thought I had was AWS Athena. But since it’s going to cost something like $2.50 USD per query for that dataset I quickly looked for an alternative.
- My answer to this was another simple go program to pull the files down from S3 then store them in a tar file. I could then process that file over and over. The process itself is done though very ugly go program to process the tar file so I could re-run my questions without having to trawl S3 over and over.
- However after time I chose not to use AWS in the end because of cost.
- So were someone to do this from scratch using the same method I eventually went with it would cost under $100 USD to redo the same calculations
- Here's Facebook's Networking @Scale 2019 recap: "This year’s conference focused on a theme of reliable networking at scale. Speakers talked about various systems and processes they have developed to improve overall network reliability or to detect network outages quickly. They also shared stories about specific network outages, how they were immediately handled, and some general lessons for improving failure resiliency and availability." You might like: Failing last and least: Design principles for network availability; BGP++ deployment and outages; What we have learned from bootstrapping 1.1.1.1; Operating Facebook’s SD-WAN network; Safe: How AWS prevents and recovers from operational events.
- 25 Experts Share Their Tips for building Scalable Web Application: Tip #1: Choosing the correct tool with scalability in mind reduces a lot of overhead; Tip #2: Caching comes with a price. Do it only to decrease costs associated with Performance and Scalability; Tip #3: Use Multiple levels of Caching in order to minimize the risk of Cache Miss.
- Google's approach requires 38x more bandwidth than a Websocket + delta solution, and delivers latencies that are 25x higher on average. Google - polling like it's the 90s.
- Google strangely chose HTTP Polling. Don’t confuse this with HTTP long polling where HTTP requests are held open (stalled) until there is an update from the server. Google is literally dumb polling their servers every 10 seconds on the off-chance there’s an update. This is about as blunt a tool as you can imagine.
- Google’s HTTP polling is 80x less efficient than a raw Websocket solution. Over a 5 minute window, the total overhead uncompressed is 68KiB vs 16KiB for long polling and a measly 852 bytes for Websockets
- The average latency for Google’s long polling solution is roughly 25x slower than any streaming transport that could have been used.
- Every request, every 10 seconds, sends the entire state object...Over a 5 minute window, once the initial state is set up, Google’s polling solution consumes 282KiB of data from the Google servers, whereas using Xdelta (encoded with base-64) over a Websocket transport, only 426 bytes is needed. That represents 677x less bandwidth needed over a 5 minute window, and 30x less bandwidth when including the initial state set up.
- The 8base tech stack: we chose Amazon Web Services (AWS) as our computing infrastructure; serverless computing using AWS Lambda; AWS Aurora MySQL and MongoDB Atlas as databases; AWS S3 (Simple Storage Service) for object storage service; AWS's API Gateway; 8base built an incredibly powerful GraphQL API engine; React; Auth0.
- The rise of the Ctaic family of programming languages. Altaic: Rise and Fall of a Linguistic Hypothesis. Interesting parallels with the lineage of programming languages. Spoken languages living side by side for long periods of times come to share vocabulary and even grammar, yet they are not part of the same family tree. Words transfer sidewise between unrelated languages rather than having a parent child relationship. I don't even know if there is formal programming language lineage chart, but it does seem languages tend to converge over time as user populations agitate for the adoption of language features from other languages into their favorite language. Even after years of principled objection to generics being added to Go, many users relentlessly advocate for Go generics. And though C++ is clearly in parent child relationship with C, over the years C++ has adopted nearly every paradigm under the sun.
- Orchestrating Robot Swarms with Java:
- How does a busy-loop go? Here's that Real-timeEventScheduler that I didn't show you before, this time with a busy-loop implemented in it. Similar to our discrete-event, we have our TimeProvider, this time it's probably the system TimeProvider. I've got the interface here and we have our queue of events. Rather than iterating or looping on our queue while we have tasks, we loop around forever and we check, is this event due to scheduled now, or basically has current time gone beyond the time my event history to be scheduled? If it is, then we run the event. Otherwise, we loop around. What this is basically doing is going, "Do I have any work? If I have some work, execute it. If not, loop back around. Do I have any work?" till it finds some work and it does it. Why did we do this? What sort of benefits does this get us? Some of the advantages that we saw is that our latency for individual events went down from basically around 5 milliseconds to effectively 0, because you're not waiting for anything to wake up, you're not waiting for a thread to get created, you're just there constantly polling, and as soon as you've got your event, you can execute it. We saw in our system that throughput events went up by three times, that's quite good for us.
- we have some parts of our computation which can be precomputed in advance. Our application startup time, we can take all these common parts of calculations and eagerly calculate them and cache the results. What that means is, when we come to communicating with our robots, we don't have to do full computation in our algorithms, we only have to do the smallest amount of computation based on what the robot is telling us
- To reduce garbage collection overhead: Remove Optional from APIs that are heavily used us, use for-loops instead of the Streams API, use an array backed data structure instead of something like HashSet or LinkedList and avoid primitive boxing, especially in places like log lines. The thing that these all have in common is basically excess object creation.
- ZGC is new in Java 11, labeled as experimental, but it's promising some seriously low pause times, in order like 10 milliseconds, on heaps of over 100 gigabytes. By just switching to ZGC, that 50 milliseconds is all the way over here, it's beyond the 99th percentile. That means less than 1 in 100 pauses are greater than 50 milliseconds and for us, that's amazing.
- It would be hard to find a better example of the risks of golden path test and development. New Cars’ Pedestrian-Safety Features Fail in Deadliest Situations. Somewhere in the test matrix should be regression tests for detecting pedestrians at night. We really need a standardized test for every autonomous car software update. It might even be a virtual course. They key is cars are not phones or DVRs. A push based over-the-air update process means a once safe vehicle is one unstable point release away from causing chaos on the road—or the death of a loved one. What if iOS 13 was the software that ran your car? Not a pretty thought.
- CockroachDB offer the lowest price for OLTP workloads, it does so while offering the highest level of consistency. Just How “Global” Is Amazon Aurora? CockroachDB would like you to know Aurora is not all that. Why? 1) It's optimized for read-heavy workloads when write scalability can be limited to a single master node in a single region. 2) Replication between regions is asynchronous, there is the potential for data loss of up to a second (i.e., a non-zero recovery point objective – RPO), and up to a minute to upgrade the read node to primary write node (i.e., 1 minute for the recovery time objective – RTO). 3) Multi-master - there is no option to scale reads to an additional region. 4) Multi-master - doubling of the maximum write throughput is gained at the expense of significantly decreased maximum read throughput. 5) Aurora multi-master does not allow for SERIALIZABLE isolation. 6) Depends on a single write node for all global writes. 7) Performs well in a single region and is durable to survive failures of an availability zone. However, there can be latency issues with writes because of the dependence on a single write instance. The distance between the client and the write node will define the write latency as all writes are performed by this node. 8) Does not have the ability to anchor data execution close to data.
- Scaling the Hotstar Platform for 50M:
- One of our key insights from 2018 was that auto-scaling would not work, which meant that we had static “ladders” that we stepped up/down to, basis the amount of “headroom” left.
- Our team took an audacious bet to run 2019 on Kubernetes (K8s). It became possible to think of building our own auto-scaling engine that took into account multiple variables that mattered to our system.
- We supported 2x more concurrency in 2019 with 10x less compute overall. This was a 6–8 month journey that had it’s roots in 2–3 months of ideation before we undertook it. This section might make it sound easy, it isn’t.
- Your system is unique, and it will require a unique solution.
- We found a system taking up more than 70% compute for a feature that we weren’t even using.
- We hear a lot about bad crypto, but what does good crypto even look like? Who talks about that? Steve Gibson, that's who. In the The Joy of Sync Steve describes how Sync.com works and declares it good: Zero-knowledge, end-to-end encryption ● File and file meta data is encrypted client-side and remains encrypted in transit and at rest. ● Web panel, file sharing and share collaboration features are also zero-knowledge. ● Private encryption keys are only accessible by the user, never by Sync. ● Passwords are never transmitted or stored, and are only ever known by the user....A randomly generated 2048 bit RSA private encryption key serves as the basis for all encryption at Sync. During account creation, a unique private key is generated and encrypted with 256 bit AES GCM, locked with the user’s password. This takes place client-side, within the web browser or app. PBKDF2 key stretching with a high iteration count is used to help make weak passwords more cryptographically secure. Encrypted private keys are stored on Sync’s servers, and downloaded and decrypted locally by the desktop app, web panel or mobile apps after successful authentication. At no time does Sync have access to a user’s private key. And there's much more about how good crypto works.
- Lyft on Operating Apache Kafka Clusters 24/7 Without A Global Ops Team. Kind of an old school example of how to run your own service and make it reliable for you. Also an example of why the cloud isn't magic sauce. Things fail and don't fix themselves. That's why people pay extra for managed services. But Lyft build the monitoring and repair software themselves and now Kafka runs without a huge operational burden.
- This could help things actually become the internet of things. Photovoltaic-powered sensors for the “internet of things”: Perovskite [solar] cells, on the other hand, can be printed using easy roll-to-roll manufacturing techniques for a few cents each; made thin, flexible, and transparent; and tuned to harvest energy from any kind of indoor and outdoor lighting. The idea, then, was combining a low-cost power source with low-cost RFID tags, which are battery-free stickers used to monitor billions of products worldwide. The stickers are equipped with tiny, ultra-high-frequency antennas that each cost around three to five cents to make...enough to power up a circuit — about 1.5 volts — and send data around 5 meters every few seconds.
Soft Stuff:
- Programming with Bigmachine is as if you have a single process with “cloudroutines” across a large cluster of physical nodes. Bigmachine (article): is an attempt to reclaim programmability in cloud computing. Bigmachine is a Go library that lets the user construct a system by writing ordinary Go code in a single, self-contained, monolithic program. This program then manages the necessary compute resources, and distributes itself across them. No infrastructure is required besides credentials to your cloud provider. Bigmachine achieves this by defining a unified programming model: a Bigmachine binary distributes callable services onto abstract “machines”. The Bigmachine library manages how these machines are provisioned, and transparently provides a mutually authenticated RPC mechanism.
- @marius: When we built Reflow, we came upon an interesting way to do cluster computing: self-managing processes. The idea was that, instead of using complicated cluster management infrastructure, we could build a vertically integrated compute stack. Because of Reflow’s simplified needs, cluster management could also be a lot simpler, so we built Reflow directly on top of a much lower level interface that could be implemented by EC2 (or really any VM provider) directly. Bigmachine is this idea reified in a Go package. It defines a programming model around the idea of an abstract “machine” that exposes a set of services. The Bigmachine runtime manages machine creation, bootstrapping, and secure RPC. Bigmachine supports any comms topology. Bigmachine also goes to great lengths to provide transparency. For example, standard I/O is sent back to the user; Go’s profile tooling “just works” and gives you profiles that are merged across the whole cluster; stats are automatically aggregated.
- @everettpberr: It’s hard to believe a gap like this exists in cloud computing today but it’s absolutely there. We deal with this every week. If I have a local program that now I want to execute across a lot of data - there’s _still_ a lot of hassle involved. BigSlice may solve this.
- Bigslice: a cluster computing system in the style of Spark. Bigslice is a Go library with which users can express high-level transformations of data. These operate on partitioned input which lets the runtime transparently distribute fine-grained operations, and to perform data shuffling across operation boundaries. We use Bigslice in many of our large-scale data processing and machine learning workloads.
- @marius: Bigslice is a distributed data processing system built on top of Bigmachine. It’s similar to Spark and FlumeJava, but: (1) it’s build for Go; (2) it fully embodies the idea of self-managing serverless computing. We’re using Bigslice for many of our large scale workloads at GRAIL. Because Bigslice is built on top of Bigmachine, it is also fully “self-managing”: the user writes their code, compiles a binary, and runs it. The binary has the capability of transparently distributing itself across a large ad hoc cluster managed by the same runtime. This model of cluster computing has turned out to be very pleasant in practice. It’s easy to make modifications across the stack, and from an operator’s perspective, all you need to do is bring along some cloud credentials. Simplicity and transparency in cloud computing.
- cloudflare/quiche: an implementation of the QUIC transport protocol and HTTP/3 as specified by the IETF. It provides a low level API for processing QUIC packets and handling connection state.
Pub Stuff:
- Numbers limit how accurately digital computers model chaos: Our work shows that the behaviour of the chaotic dynamical systems is richer than any digital computer can capture. Chaos is more commonplace than many people may realise and even for very simple chaotic systems, numbers used by digital computers can lead to errors that are not obvious but can have a big impact. Ultimately, computers can’t simulate everything.
- The Effects of Mixing Machine Learning and Human Judgment: Considered in tandem, these findings indicate that collaboration between humans and machines does not necessarily lead to better outcomes, and human supervision does not sufficiently address problems when algorithms err or demonstrate concerning biases. If machines are to improve outcomes in the criminal justice system and beyond, future research must further investigate their practical role: an input to human decision makers.