Hot Trend: Move Behavior to Data for a New Interactive Application Architecture
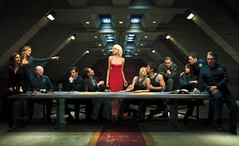
Two forces account for the trend of moving behavior to data: larger values used in key-value stores and spotty cloud networks. For some time we've seen functions pushed close to data with MapReduce, which is a batch process, but we are now seeing this model extend to interactive applications, which match the current emphasis on highly scalable, real-time, event driven applications.
To see the trend look at the increasing support for collocated behavior at the datastore level:
- HBase is implementing a coprocessor feature.
- Cassandra is also implementing coprocessors or plugins.
- Membase is creating NodeCode, which is a process for executing functions on data in the database.
- MongoDB supports JavaScript stored procedures.
- VoltDB has Java based stored procedures.
- In-memory DataGrid products like GigaSpaces have been doing something like this forever. The key difference historically being GigaSpaces' tight integration with languages C++ and Java, instead of using a language agnostic protocol based approach.
- Redis implements a set of data structures on the server side, which are essentially amazingly useful built-in stored procedures which have become the basis for higher level primitives for applications.
- Google has blessed the trend with the trigger functionality in Percolator, a system for incrementally processing updates to a large data set.
Isn't this Just Stored Procedures All Over Again?
But wait I hear you say, we've always had stored procedures in databases for this exactly the same efficiency reasons. Why is this happening all over again? Well, to really understand that you'll need to watch the entire Battle Star Galactica series. But, in short: 1) the move to the cloud, 2) large values, 3) stored procedures are now horizontally scalable and won't become the bottleneck, 4) more palatable implementation languages (PL/SQL anyone?).
The Problem of the Cloud, Spotty Networks, and Large Values
For the past decade or so, to overcome non-scalable databases, objects were put in caches. The caches were sharded, or spread horizontally in order to scale. The logic tier of an application was also scaled horizontally across nodes, using the caching tier for fast and scalable data access. An application does a get, processes the data, and does a set with the new values. This works great, until...
...until you move to the cloud and you no-longer have direct control over the quality of your network. One of the biggest complaints about the Amazon cloud is its poor network. Many developers have found Amazon's network lossy, with a high and variable latency. Connections consistently drop which has caused a number to consider moving out of the cloud to get more control over the network.
Spotty networks are also exacerbated by large values. With the move to key-value stores we've seen values become larger as they are denormalized. Values have become fatter as more and more references have been transformed into contained objects. A user object, for example, may now contain its social network. If you have 10,000 people in your social network that user object just became large. And this trend is accelerating. These large objects over a spotty network are a problem. One partial solution is for databases to support programmers with easier to use references.
When you control your own equipment you can of course use powerful SANs, SSDs, fast fibre channel connections, more disks, more spindles, write caches, smart controllers, and so on. If you've made the move to the cloud however, these tricks are not available to you, so you have to make do with the resources that are available.
Sharding Could Remove Stored Procedures as the Bottleneck
Another solution is to move the functionality that was in the logic tier to the database tier. Won't this become a bottleneck as it always did before? Not necessarily. The difference now is that databases themselves are sharded, which means the functions running in the database may not become the bottleneck.
Stored procedures in the database bring up all sorts of locking and threading issues that could kill performance. The clean get/set a buffer from a slab type logic is certainly complicated by having application logic running at the same time. MongoDB takes a giant lock while a stored proc executes. Membase is getting around this somewhat by moving the code to a separate process and leaving the data manger clean, which of course adds another hop. VoltDB puts stringent requirements on how long a stored procedure can operate.
This trend is why in Troubles With Sharding - What Can We Learn From The Foursquare Incident? I defined sharding as: The goal of sharding is to continually structure a system to ensure data is chunked in small enough units and spread across enough nodes that data operations are not limited by resources. The trick is to keep stored procedures within bounds by sizing shards not simply on memory, but on CPU and IO as well. A little different approach than we have now, but one that may be necessary if data and behavior are to be collocated.
The Server Side Environment Needs to be Richer
Another weakness of these systems is that although they will have nicer server side languages (Java, JavaScript, etc), the programming model on the server side is still impoverished. It would great to see something like Node.js merged in with the database so programmers would have a real application platform to build on.
An obvious question is how should application logic be divided? By being able to shard on multiple criteria the typical arguments against using the database as an application container are muted a bit because the same horizontal partitioning at the application layer can be pushed down to do the database. Given this, should all application logic be pushed into the database? Should some reside in the database and some outside? How do you know where the line is? Should a web server be merged into each shard so clients can directly access code via REST? Could or should this replace the service tier? How does this work with search, secondary indexes and other database oriented features? It's these kind of difficult to answer questions that still make keeping behavior out of the database very attractive.
What Does All this Seem to Add Up To?
The next generation cloud enabled, interactive application architecture may be:
- A rich, asynchronous, evented server side application environment, coded in a dynamic language with direct, low latency access to a collocated and integrated datastore.
- Dynamically sharded to ensure both scalable data and behavior.
Or maybe that's just "Silly me. Silly, silly me."