Stuff The Internet Says On Scalability For December 8th, 2017
Hey, it's HighScalability time:
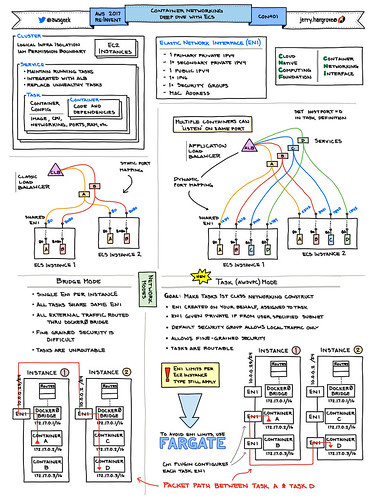
AWS Geek creates spectacular visual summaries.
If you like this sort of Stuff then please support me on Patreon. And please recommend my new book—Explain the Cloud Like I'm 10—to those looking to understand the cloud. I think they'll like it.
- 127 terabytes: per year growth in blockchain if bitcoin wins; 4: hours from tabula rasa to chess god; 1.4 billion: Slack jobs per day; 400: hyperscale data centers worldwide by 2018; 9.8X: Machine Learning Engineer job growth; 14%: Ethereum transactions are for Cryptokitties; 80: seconds per hash on 55 year old IBM 1401 mainframe; $110 billion: app stores spending in 2018; 25: years since first text message; 4,000: AWS code pushes per day; two elephants: of space dust hits earth every day; 70%: neural nets outperformed cache-optimized B-Trees while saving an order-of-magnitude in memory;
- Quotable Quotes:
- @DavidBrin: Now that's what I call engineering! [Voyager 1] Thrusters that haven't been used in 37 years - still reliable!
- drkoalaman: So despite not supporting other cryptos the majority of my time on the DNM's I think its officially time to step away from bitcoin, at least for the time being. Went to do a direct deal today with a vendor, realized my $250 purchase would end up costing me $315 or so with fees and would still take probably 24 hours to get to him. As of this morning the lowest electrum fee was approx $32 to send coin.... and people reporting at the highest level still not having coin move 12-16 hours later. Vendors are loving this surge but its creating a sellers market and backlogging the blockchain and fees are just crazy... Not to mention not knowing if your $250 will be worth $300 when it gets to the vendor or a random drop in BTC causing it to be less...
- Alex Lindsay: 30 years ago you couldn’t get cash on Sunday. Now you can send cash on your watch.
- @prestonjbyrne: “We’re launching on Ethereum” == “100% uptime, unless someone makes a cat app, at which point all bets are off”
- @GossiTheDog: So I got somebody to talk, without names, about one of the big S3 bucket leaks. A developer set a bucket to open by mistake. They had open S3 bucket monitoring scripts running and got warning emails, which nobody did anything with - nobody had ownership of S3 buckets.
- @jaksprats: reInvent 2017 Amazon Time Sync Service … Prediction: by reInvent 2018 either they build their own Spanner or they acquire CockroachDB
- @PatrickMcFadin: 4/ I don’t think we’ll see many more big AWS database announcements after this year. What they have is “good enough” for them and the consensus is they are moving to AI and “everything Alexa” quickly.
- Eric Horvitz~ in 50 summers, the aviation industry went from canvas flopping on a beach to the Boeing 707...And what is this thing called consciousness, that we use the word consciousness to refer to. Where does that come from? What are these subjective states? We have no idea. We have theories and reflections, but they are not really based in any scientific theories just yet. However, is it possible in 50 summers, we have a whole new world. We have big surprises. We understand how minds work.
- @martinkl: Google Realtime API is shutting down … — It’s so risky to rely on proprietary services for building apps.
- @jeremiahg: Equifax’s stock price isn’t recovering post-breach as expected. If the stock remains flat over the next two months, it’ll been interesting to discuss why — what made them different.
- @xmal: A possible solution to the Fermi Paradox is that any sufficiently advanced civilization is dedicating all its resources to bitcoin mining.
- @rbhar90: The AI future where megacorporations control enormous datasets and near infinite compute letting them machine learn to predict our every action terrifies me. At NIPS, it's clear this future is nearer rather than further.
- Sue Hartley: This plant has built this little structure. It's sort of like a barracks for the ant army. And they live inside. When herbevores arrive the ant army comes out and attacks the herbivores...The plant can spend up to 20% of its resources housing and feeding its army.
- Netflix: With great elasticity comes great responsibility.
- Michael Widmann: There’s a change in the (NATO) mindset to accept that computers, just like aircraft and ships, have an offensive capability. I need to do a certain mission and I have an air asset, I also have a cyber asset. What fits best for the me to get the effect I want?
- tomswartz: Most commonly, I use my SDR [Software Defined Radio] for: - Listening to ham (amateur) bands - Receiving NOAA/Meteor satellite images - Airplane tracking. There are quite a few other use cases
- nutrecht: Part of a microservice architecure is a mature automated CI/CD pipeline. It's a hard demand. If you don't have the maturity of a company to have automated releases you don't have the maturity to try to pull off microservices.
- @cloud_opinion: Typically a bar wants you doing shots after few drinks - shots tend to be more expensive and you are too drunk to know. I just explained serverless pricing to you in terms you can understand.
- biocomputation: Microservices are just a cargo cult. Most of these companies have a few thousand customers at most, and could scale by running multiple copies of their application on different servers. The real lesson here is to write modular apps that aren't bloated resource pigs. Otherwise scaling is even more difficult and expensive.
- @mweagle: Containers: the create once, debug everywhere packaging format.
- @greengart: Qualcomm says we are "in the era between G's" (translation: 4G wireless networks have been deployed, 5G isn't ready yet) #snapdragonsummit
- sisyphus: I love this new trend of adopting service architectures way before they are needed because it's fashionable, then 'solving' this particular overhead by centralizing your distributed version control. Next up: 'our monorepo wastes too much time and bandwidth and disk space pulling down history for things that are not relevant to our team, how we did partial checkouts to further reinvent svn on top of git'
- Jani Tarvainen: All the results clearly display that there is a price to pay for server side rendered React.js. This is no surprise as vDOM template rendering is more complex than string based processing. In this light the performance of ReactDOMServer with optimisations is downright impressive.
- Robert M. Sapolsky: The cortex and limbic system are not separate, as scads of axonal projections course between the two. Crucially, those projections are bidirectional—the limbic system talks to the cortex, rather than merely being reined in by it. The false dichotomy between thought and feeling is presented in the classic Descartes’ Error, by the neurologist Antonio Damasio
- Rodney A. Brooks: The field principle. The first pillar is the assumption that nature is made of fields. A field is a set of physical properties that exist at every point of space.
- Andrei Frumusanu: The new Snapdragon 845 brings with itself one of the biggest architectural shifts in the ARM SoC space with the first implementation of the new DynamIQ cluster hierarchy. With an expected solid 30% performance boost on both CPU and GPU we're likely to see a healthy upgrade for 2018 flagship devices.
- semiengineering~ spending on traditional data center infrastructure dropped 18% between 2015 and 2017, while spending on infrastructure products for the public cloud rose 35%. Much of that change reflects and ongoing migration of enterprises to the cloud. The percentage of workloads running on public-cloud platforms will rise to 60% by 2019, compared with 45% today. During 2016, collectively, Amazon, Microsoft and Google parent company Alphabet spent $31.54 billion in capital costs and leases, up 22% from the year before.
- @ahmetb: This guy heated up his RAM with a heat gun to debug a @golang runtime bug. I hereby nominate him to most persistent Gopher of the year award.
-
@jeffbarr: Power users of #AWS (Lambda + S3 + Batch + CloudFront) - "Using this setup on AWS Batch, we are able to generate more than 3.75 million tiles per minute and render the entire world in less than a week!"...
-
@tommorris: Basically every response to "Bitcoin mining uses up huge amounts of energy" I've seen can be categorised either as shitty economics, global warming denial, or whataboutism about the cost of minting metal coins (ignoring that most non-crypto transactions are electronic via banks).
-
polyomino: They would have to tumble the bitcoin so that the sale is not linked to their bitcoin. Then, they can cash it out by trading it in an exchange and withdrawing usd to their bank, or they can trade it for cash on something like localbitcoins.com. The Bitcoin/usd market is liquid enough to absorb this amount of money.
-
@cmeik: I'll be honest, when someone slams Erlang for not scaling to over 100 nodes it really burns me because they are most likely programming in a language without a primitive for sending between nodes.
-
@cloud_opinion: There is a distinct moment that doomed Openstack - it was argument about AWS API compatability - hearing that Kubecon is at this critical juncture on a specific topic. No spoilers yet 😀 #KubeCon
-
melissa mcewen: You don’t even need React: come on, I mean most of you are building simple disposable sites that won’t even be around in a year. Do you really need to build that brochure site in React?
- flomotlik: With Fargate and AppSync out is it time to stop using API Gateway and Lambda for API Backends? Imho API Gateway and Lambda was always pretty cumbersome to set up, not just because of the setup but also because of the code separation (one large lambda, many small lambdas, …). With Fargate being basically Serverless containers and AppSync as GraphQL backend what upside does API Gateway still have (with very few exceptions like combining several endpoints into one API). For any kind of standard API Backend (either REST or GraphQL) I have a really hard time seeing the upside of APIG/Lambda compared to either Fargate or AppSync. Which should probably be a solution for >90% of APIs we’re building. Fargate of course combined with Application Load Balancers
-
François Chollet: If intelligence is fundamentally linked to specific sensorimotor modalities, a specific environment, a specific upbringing, and a specific problem to solve, then you cannot hope to arbitrarily increase the intelligence of an agent merely by tuning its brain — no more than you can increase the throughput of a factory line by speeding up the conveyor belt
-
Timothy Morgan: Something very profound is going on at Dell...We suspect that more than a few hyperscalers and cloud builders are buying machines from Dell’s custom server business unit...In the quarter ended in September, Dell grew its server unit shipments by 11.2 percent to 503,000 machines...So Dell must be selling a lot of beefy machines to someone. To a lot of someones. And moreover, Dell’s average selling price is up by around 24 percent, and that probably means it sold a lot of really cheap boxes and a lot of really expensive ones, perhaps laden with GPU and FPGA accelerators and lots of memory.
-
jandrewrogers: As someone who waited for the new [Skylake] server CPUs, the microarchitecture made a few changes that should substantially improve its performance for data-intensive workloads: - 6 memory channels (instead of 4) - new CPU cache architecture that should show big gains for things like databases - new vector ISA (AVX-512) that is significantly more useful than AVX2, in addition to being twice as wide. The first two should be instant wins for things like databases. AVX-512 isn't going to be used in much software yet but it is arguably the first broadly usable vector ISA that Intel has produced. This should enable some significant performance gains in the future as code is rewritten to take advantage of it. (Not idle speculation on the latter, we're queued up to get some of this hardware for exactly this purpose. Previously vectorizing wasn't worth the effort outside of narrow, special cases but AVX-512 appears to change that.)
-
leetbulb: I built a fairly large system using the Serverless "framework" on AWS. It's been running for over 8 months now with zero maintenance and zero service disruptions, with an ingress of ~50M events per day and providing a sleek ReactJS/SemanticUI frontend that the users seem to really enjoy. Being a side project, it's been relatively stress-free. While the application is still very profitable, the cost is ~10x its implementation on traditional servers. I'm not going to argue the pro's and con's, just providing some numbers. At this point, we're working to optimize the original stack in order to reduce costs. I will say though...honestly, working with Serverless and the AWS stack is a very pleasant experience. The primary cost (~60%) is due to the high volume of API Gateway requests.
-
foobarbazetc: I’ve been involved in various Postgres roles (developer on it, consultant for it for BigCo, using it at my startup now) for around 18 years. I’ve never in that time seen a pacemaker/corosync/etc/etc configuration go well. Ever. I have seen corrupted DBs, fail overs for no reason, etc. The worst things always happen when the failover doesn’t go according to plan and someone accidentally nukes the DB at 2am. The lesson I’ve taken from this is it’s better to have 15-20 minutes of downtime in the unlikely event a primary goes down and run a manual failover/takeover script then it is to rely on automation. pgbouncer makes this easy enough.
-
pmoriarty: Whenever I hear about people spending outrageous amounts of money for something that should be really cheap [virtual cats on the Ethereum blockchain], the first explanation that springs to mind is money laundering.
-
Danny Lange: From a strict technical perspective, we always look for the rewards function that drives the machine . . . The rewards function in an Amazon system is, get the customer to click the purchase button. At Netflix, it’s get the customer to click on one of our TV shows. What is the rewards function of a drone? Find the bad guys and eliminate them . . . It’s really what you define as the end goal of the system [that matters].
-
@EricHolthaus: Uhhh... about bitcoin... it's actually ruining the planet. The bitcoin computer network currently uses as much electricity as Denmark. In 18 months, it will use as much as the entire United States. Something's gotta give. This simply can’t continue.
-
@damonedwards: Watching @adrianco speak at #kubecon thinking about how quickly the standard units of deployment have changed. Machine images, containers, functions... guess what isn’t there? app packages (Yes he mentioned bare metal instances, but that “old way” now feels like edge cases only)
-
boyter: This [Serverless Aurora] was also the biggest news of re:Invent for me. Total game changer. No need to provision large R instance types to support the batch processing that happens a 3AM and sits idle most of the time. All environmental tiers can have 100% the same configuration. I know of some projects burning millions a month in database costs because they like to replicate environments for testing branches. Switching to serverless Aurora would reduce that cost to probably low thousands.
-
Eric Horvitz: About 125 years or so of rich studies of cognition have revealed biases and blind spots and limitations in all human beings. That’s part of our natural human substrate of our minds. Could we build systems that understand in detail and in context the things that you’ll forget. Your ability to do two things at once and how to help you balance multi-tasking for example. Understanding how you visualize, what your learning challenges might be in any setting. But what you consider hard. Why is that math concept hard to get? You can imagine, systems that understand us so deeply someday, that they beautifully complement us. They know when to come forward. They are almost invisible. But they extend us. Now part of the trick there is not just complementarity, it’s coordination. When. How does a mix of initiatives work where a machine will do one thing and then a human takes over.
- Will AI kill database indexes too? Paper Summary. The Case for Learned Index Structures: The paper [by Tim Kraska, Alex Beutel, Ed Chi, Jeff Dean, Neoklis Polyzotis] aims to demonstrate that "machine learned models have the potential to provide significant benefits over state-of-the-art database indexes". If this research bears more fruit, we may look back and say, the indexes were first to fall, and gradually other database components (sorting algorithms, query optimization, joins) were replaced with neural networks (NNs)...The paper shows that by using NNs to learn the data distribution we can have a graybox approach to index design and reap performance benefits by designing the indexing to be data-aware.
- Smooth Sailing with Kubernetes. A truly beautiful comic by Scott McCloud on "Learn about Kubernetes and how you can use it for continuous integration and delivery." That takes some real skill. Oh, and I guess you might learn something too.
- How do you create a scalable software organization that avoids the inevitable limitations imposed by top-down control? Chaos engineering. Chaos engineering enforces an architecture without system architects. By continuously running chaos tests you are requiring conformation to a SLA without the bureaucracy. The system is held in check by an algorithm. AWS re:invent 2017: A Day in the Life of a Netflix Engineer III.
- Excellent example of how to upgrade an existing system by looking at what went wrong with the old, avoiding the dreaded rewrite, and creating a successful roll out plan. Scaling Slack’s Job Queue: On our busiest days, the system processes over 1.4 billion jobs at a peak rate of 33,000 per second...about a year ago, Slack experienced a significant production outage due to the job queue. Resource contention in our database layer led to a slowdown in the execution of jobs, which caused Redis to reach its maximum configured memory limit. At this point, because Redis had no free memory, we could no longer enqueue new jobs, which meant that all the Slack operations that depend on the job queue were failing...We thought about replacing Redis with Kafka altogether, but quickly realized that this route would require significant changes to the application logic...we decided to add Kafka in front of Redis rather than replacing Redis with Kafka outright...we developed Kafkagate, a new stateless service written in Go, to enqueue jobs to Kafka...JQRelay is a stateless service written in Go that relays jobs from a Kafka topic to its corresponding Redis cluster...Our cluster runs the 0.10.1.2 version of Kafka, has 16 brokers and runs on i3.2xlarge EC2 machines. Every topic has 32 partitions, with a replication factor of 3, and a retention period of 2 days. We use rack-aware replication (in which a “rack” corresponds to an AWS availability zone) for fault tolerance and have unclean leader election enabled...Double writes: We started by double writing jobs to both the current and new system.
- Get your own copy of the C++ 17 standard. And here's a Meeting C++ 2017 Trip Report. Followed by a Summary of C++17 features.
- Anyone ever go from microservices to a monolith? Why, yes. thedarkwolf: The company had originally wanted to build microservices because some manager read a blog post or something. But the organization was really not set up to support microservices. They had a very elaborate production deployment process with layers and layers of approvals. As a result, they would just deploy all of the "microservices" at once every few months. They also did a poor job implementing the microservice patterns in general. Also, this application only had about 500 users. Refactoring back into a monolith simplified the code, simplified the deployment process, and made dev setup so much easier. That's not to say microservices are bad in general, but they are not good for every project or for every organization. Also, The Microservices Misconception.
- A semiotic approach to Public Cloud Services Comparison.
- Apple's approach to machine learning is device centric, based on the idea of differential privacy. What is that? Thanks to an Apple that now cracks the door open just a smidge, we can now know. Learning with Privacy at Scale (pdf). Differential privacy: provides a mathematically rigorous definition of privacy and is one of the strongest guarantees of privacy available. It is rooted in the idea that carefully calibrated noise can mask a user’s data. When many people submit data, the noise that has been added averages out and meaningful information emerges...Our system architecture consists of device-side and server-side data processing. On the device, the privatization stage ensures raw data is made differentially private. The restricted-access server does data processing that can be further divided into the ingestion and aggregation stages.
- More reinvent experience reports. AWS re:Invent 2017 Recap: Kubernetes, Security, and Microservices. Glenn’s Take on re:Invent 2017 - Part 3. Glenn’s Take on re:Invent Part 2. re:Invent 2017 Serverless Recap. AWS re:Invent Day 2 Keynote Announcements: Alexa for Business, Cloud9 IDE & AWS Lambda Enhancements. re:Invent 2017 security review: The big theme for new videos this year was a focus on using multiple AWS accounts; GuardDuty (video). This service monitors your CloudTrail logs, VPC flow logs, and DNS logging; Cross-region VPC peering: This is huge. Previously, if you had two regions that you wanted to talk to each other, you either needed to expose services in those region publicly;
- Use a sledge hammer not a magnet when you want to make really really sure your hard drive is dead dead dead. Hard Drive Destruction.
- We have a new entrant into the up and coming CRDT database market. AntidoteDB: Antidote is an artifact of the SyncFree Project. It incorporates cutting-edge research results on distributed systems and modern data stores, meeting for your needs! Antidote is the first to provide all of these features in one data store: Transactional Causal+ Consistency, Replication across DCs, Horizontal scalability by partitioning of the key space, Operation-based CRDT support, Partial Replication. Also, Kuhiro.
- What I learned from doing 1000 code reviews: Throw an exception when things go wrong; Use the most specific type possible; Use Optionals instead of nulls; “Unlift” methods whenever possible.
- Some cost saving opportunities you may have missed in the flurry of AWS announcements. Six new features that help reduce AWS spend announced at reInvent 2017. AWS Fargate: By carefully configuring compute and memory resource requirements for containers potential reduction is cost is possible. EC2 M5 Instances: Those that currently use M4 family instances can save 4% by switching to M5 family instances. EC2 H1 Instances: applications that use D2.xlarge (6 TB minimum) could switch to H1.2xlarge and get twice the compute power, similar memory and 2TB storage space and reduce cost by 20%. Hibernation for Spot Instances: This new feature offers potentially significant cost reduction by moving stateful, time insensitive applications that may be running on on-demand/reserved instances to spot instances. Savings can be in the range of 70%-90%. New Spot Pricing Model and Spot on RunInstances: It is possible that applications that use spot instance may benefit from reduced volatility and may see reduced cost. Amazon Aurora Serverless: could potentially reduce RDS cost significantly, as the pricing is based on the actual usage.
- Here's the creative way Etsy handles hot keys in their cache system. How Etsy caches: hashing, Ketama, and cache smearing: We use a technique I call cache smearing to help with hot keys. We continue to use consistent hashing, but add a small amount of entropy to certain hot cache keys for all reads and writes. This effectively turns one key into several, each storing the same data and letting the hash function distribute the read and write volume for the new keys among several hosts...Cache smearing (named after the technique of smearing leap seconds over time) trades efficiency for throughput, making a classic space-vs-time tradeoff. The same value is stored on multiple hosts, instead of once per pool, which is not maximally efficient. But it allows multiple hosts to serve requests for that key, preventing any one host from being overloaded and increasing the overall read rate of the cache pool.
- Videos from Neural Information Processing Systems 2017 are now available. Learning State Representations. The Unreasonable Effectiveness of Structure.
- IDLs are as old as networks. Here's a very thoughtful exploration of what they are for and why they are necessary. Thrift on Steroids: A Tale of Scale and Abstraction: I think there’s a delicate balance between providing solutions that are “easy” from a developer point of view but may provide longer term drawbacks when it comes to building complex systems the “right” way. I see RPC as an example of this. It’s an “easy” abstraction but it hides a lot of complexity. Service meshes might even be in this category, but they have obvious upsides when it comes to building software in a way that is scalable.
- Here are some Optimization for Deep Learning Highlights in 2017.
- In your world spanning database how do you make sure computation stays near data? Using “Follow-the-Workload” to Beat the Latency-Survivability Tradeoff in CockroachDB: if a leaseholder located in the U.S. receives a request from a node located in Australia, the request has to travel halfway around the world (and back) before processing, adding 150-200ms of network latency. On the other hand, if the leaseholder was located in Australia, processing this same request would result in single digit milliseconds...With “follow-the-workload”, segmentation can be accomplished by both time, as described in “follow-the-sun”, and by primary key. Primary keys can be established based upon a user’s location (e.g. state or country) and further used to pre-split tables. If DBAs decide to do this, CockroachDB will employ “follow-the-workload” to ensure that the lease for a given range will be located nearest the state that its users originate requests from.
- As much as it can be. Quantum Computing Explained. Here's something else difficult to understand. A Gentle Introduction to Erasure Codes.
- Machine learning benchmarks: Hardware providers (part 1). Compares GCE, AWS, IBM Softlayer, Hetzner. Quite a range of results. General observations: The performance of most frameworks does not scale well with additional CPU cores beyond a certain point (8 cores), even on machines with many more cores; Fewer cores with high clock speeds perform much better than high number of cores with low clock speeds. Stick to the former to cut overall costs; Dedicated servers like Hetzner can be an order of magnitude cheaper than cloud, for the high performance and free bandwidth they provide. You should definitely take this into consideration while renting machines for extended (month+) periods of use.
- From a programmer's perspective FMSs and threads are very different. FSMs can be abstractly mapped onto threads for scheduling, aync IO, load balancing, and priority. Threads are the lower level concept. Threads lack the power of explicitly stating logic. Threads are merely an implementation detail. So can't agree with JohnCarter: Everything you can implement in a collection of FSM’s, you can implement straightforwardly in a collection of an equal number of threads and vice versa.
- Deep Learning 101: Demystifying Tensors: Tensorflow and systems like it are all about taking programs that describe a machine learning architecture (such as a deep neural network) and adjusting the parameters of that architecture to minimize some sort of error value. They do this by not only creating a data structure representing our program, but they also produce a data structure that represents the gradient of the error value with respect to all of the parameters of our model. Having such a gradient function makes the optimization go much more easily...Tensors figure prominently in the mathematical underpinnings of general relativity and are fundamental to other branches of physics as well. But just as the mathematical concepts of matrices and vectors can be simplified down to the arrays we use in computers, tensors can also be simplified and represented as multidimensional arrays and some related operations. Unfortunately, things aren’t quite as simple as they were with matrices and vectors, largely because there isn’t an obvious and simple set of operations to be performed on tensors like there were with matrices and vectors. There is some really good news, though. Even though we can’t write just a few operations on tensors, we can write down a set of patterns of operations on tensors. That isn’t quite enough, however, because programs written in terms of these patterns can’t be executed at all efficiently as they were written. The rest of the good news is that our inefficient but easy to write programs can be transformed (almost) automatically into programs that do execute pretty efficiently. Also, What's a Tensor?
- A good Introduction to the Java Memory Model. Complicated? Oh yes.
- Always helps to learn from the masters. Concurrent Servers Part 5 - Redis case study: One of Redis's main claims to fame around the time of its original release in 2009 was its speed - the sheer number of concurrent client connections the server could handle. It was especially notable that Redis did this all in a single thread, without any complex locking and synchronization schemes on the data stored in memory. This feat was achieved by Redis's own implementation of an event-driven library which is wrapping the fastest event loop available on a system (epoll for Linux, kqueue for BSD and so on). This library is called ae. ae makes it possible to write a fast server as long as none of the internals are blocking, which Redis goes to great lengths to guarantee.
- There are more options than ever for solving problems in different ways. How Jet Built a GPU-Powered Fulfillment Engine with F# and CUDA: This post introduced Jet.com’s exponentially complex merchant selection problem and our approach to solving it within an environment of microservices written in F# and running in the Azure cloud. I covered implementation details of a brute force GPU search approach to merchant selection and how this algorithm is used from a RESTful microservice.
- Do databases and containers mix? Yes, but you must stir vigorously. Containerizing Databases at New Relic, Part 1: What We Learned: We now deploy all new database instances in Docker containers, regardless of their type and version. This has allowed us to reach a new level of consistency and reproducibility across our database tiers. Having a single deployment process means we can deliver new databases to internal teams faster and more reliably. Even without full resource isolation, our resource efficiency has greatly improved. We’ve also been able to add support for a new backup solution, monitoring, and operating systems across our database tiers by building new images.
- If you've ever want to understand how CPUs handle interrupts, here's a good podcast from the good folks at Embedded.fm: 224: INTERRUPTS TO INTERRUPT INTERRUPTS.
- Good explanation of Cluster Schedulers: Schedulers are not superior because they replace traditional Operations tooling with abstractions underpinned by complex distributed systems, but because, ironically, by dint of this complexity, they make it vastly simpler for the end users to reason about the operational semantics of the applications they build. Schedulers make it possible for developers to think about the operation of their service in the form of code, making it possible to truly get one step closer to the DevOps ideal of shared ownership of holistic software lifecycle.
- Another stab at explaining CQRS and event sourcing. Patterns for designing flexible architecture in node.js (CQRS/ES/Onion): This architecture divides the software into layers using a simple rule: outer layers can depend on lower layers, but no code in the lower layer can depend on any code in the outer layer.
- The Entity Service Antipattern: I contend that “entity services” are an antipattern...So, let’s look at the resulting context of moving to microservices with entity services: Performance analysis and debugging is more difficult. Tracing tools such as Zipkin are necessary. Additional overhead of marshalling and parsing requests and replies consumes some of our precious latency budget. Individual units of code are smaller. Each team can deploy on its own cadence. Semantic coupling requires cross-team negotiation. Features mainly accrue in “nexuses” such as API, aggregator, or UI servers. Entity services are invoked on nearly every request, so they will become heavily loaded. Overall availability is coupled to many different services, even though we expect individual services to be deployed frequently. (A deployment look exactly like an outage to callers!)
- Stateful Multi-Stream Processing in Python with Wallaroo: Wallaroo allows you to represent data processing tasks as distinct pipelines from the ingestion of data to the emission of outputs. A Wallaroo application is composed of one or more of these pipelines. An application is then distributed over one or more workers, which correspond to Wallaroo processes...Wallaroo provides in-memory application state. This means that we don’t rely on costly and potentially unreliable calls to external systems to update and read state...When you define a stateful Wallaroo application, you define a state partition by providing a set of partition keys and a partition function that maps inputs to keys. Wallaroo divides its state into distinct state entities in a one-to-one correspondence with the partition keys. These state entities act as boundaries for atomic transactions (an idea inspired by this paper by Pat Helland). They also act as units of parallelization, both within and between workers.
- A reminder that Cliff Click is laying down some wisdom in his new podcast series. Newest: Build Systems and Make, Cost Models, and Queuing In Practice.
- RiotGames/cloud-inquisitor: tool to enforce ownership and data security within AWS.
- mozilla/DeepSpeech: A TensorFlow implementation of Baidu's DeepSpeech architecture.
- aws/aws-amplify: a JavaScript library for frontend and mobile developers building cloud-enabled applications. The library is a declarative interface across different categories of operations in order to make common tasks easier to add into your application. The default implementation works with Amazon Web Services (AWS) resources but is designed to be open and pluggable for usage with other cloud services that wish to provide an implementation or custom backends.
- fanout/python-faas-grip: Function-as-a-service backends are not well-suited for handling long-lived connections, such as HTTP streams or WebSockets, because the function invocations are meant to be short-lived. The FaaS GRIP library makes it easy to delegate long-lived connection management to Fanout Cloud. This way, backend functions only need to be invoked when there is connection activity, rather than having to run for the duration of each connection.
- circonus-labs/libmtev: a toolkit for building high-performance servers in C.
- Ziria: A DSL for wireless systems programming: Ziria is a new domain-specific language (DSL) that offers programming abstractions suitable for wireless physical (PHY) layer tasks while emphasizing the pipeline reconfiguration aspects of PHY programming. The Ziria compiler implements a rich set of specialized optimizations, such as lookup table generation and pipeline fusion. We also offer a novel – due to pipeline reconfiguration – algorithm to optimize the data widths of computations in Ziria pipelines. Also, tomswartz07/CPOSC2017.
- Building a Mobile Gaming Analytics Platform - a Reference Architecture: This reference architecture provides a high level approach to collect, store, and analyze large amounts of player-telemetry data on Google Cloud Platform. More specifically, you will learn two main architecture patterns for analyzing mobile game events: Real-time processing of individual events using a streaming processing pattern. Bulk processing of aggregated events using a batch processing pattern
- Mastering Chess and Shogi by Self-Play with a General Reinforcement Learning Algorithm: In this paper, we generalise this approach into a single AlphaZero algorithm that can achieve, tabula rasa, superhuman performance in many challenging domains. Starting from random play, and given no domain knowledge except the game rules, AlphaZero achieved within 24 hours a superhuman level of play in the games of chess and shogi (Japanese chess) as well as Go, and convincingly defeated a world-champion program in each case.
- Stewardship in the "Age of Algorithms": This is essentially the first paper I am aware of which tries to effectively make progress on the stewardship challenges facing our society in the so-called “Age of Algorithms;” the paper concludes with some discussion of the failure to address these challenges to date, and the implications for the roles of archivists as opposed to other players in the broader enterprise of stewardship — that is, the capture of a record of the present and the transmission of this record, and the records bequeathed by the past, into the future. It may well be that we see the emergence of a new group of creators of documentation, perhaps predominantly social scientists and humanists, taking the front lines in dealing with the “Age of Algorithms,” with their materials then destined for our memory organizations to be cared for into the future.
- The Case for Learned Index Structures: Indexes are models: a B-Tree-Index can be seen as a model to map a key to the position of a record within a sorted array, a Hash-Index as a model to map a key to a position of a record within an unsorted array, and a BitMap-Index as a model to indicate if a data record exists or not. In this exploratory research paper, we start from this premise and posit that all existing index structures can be replaced with other types of models, including deep-learning models, which we term learned indexes. The key idea is that a model can learn the sort order or structure of lookup keys and use this signal to effectively predict the position or existence of records. We theoretically analyze under which conditions learned indexes outperform traditional index structures and describe the main challenges in designing learned index structures. Our initial results show, that by using neural nets we are able to outperform cache-optimized B-Trees by up to 70% in speed while saving an order-of-magnitude in memory over several real-world data sets. More importantly though, we believe that the idea of replacing core components of a data management system through learned models has far reaching implications for future systems designs and that this work just provides a glimpse of what might be possible.